AI Predictive Maintenance in Telecom Reducing Downtime Effectively
Topic: AI Research Tools
Industry: Telecommunications
Discover how AI-driven predictive maintenance can reduce downtime in telecom networks enhance reliability and improve operational efficiency for telecom companies
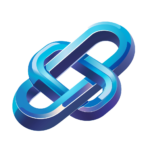
AI-Driven Predictive Maintenance: Reducing Downtime for Telecom Networks
Understanding Predictive Maintenance in Telecommunications
In the fast-paced world of telecommunications, maintaining network reliability is paramount. Downtime can lead to significant revenue loss and damage to customer trust. Predictive maintenance, powered by artificial intelligence (AI), offers a solution that not only reduces downtime but also enhances operational efficiency. By leveraging AI research tools, telecom companies can anticipate equipment failures before they occur, ensuring uninterrupted service delivery.
The Role of AI in Predictive Maintenance
AI technologies enable telecom operators to analyze vast amounts of data generated by network equipment. Through machine learning algorithms, these systems can identify patterns and anomalies that may indicate potential failures. By implementing AI-driven predictive maintenance strategies, companies can shift from reactive maintenance approaches to proactive measures, thereby minimizing unexpected outages.
Key Components of AI-Driven Predictive Maintenance
- Data Collection: The first step involves gathering data from network devices and sensors. This data includes performance metrics, environmental conditions, and historical maintenance records.
- Data Analysis: Machine learning models process the collected data to identify trends and predict future failures. These models can be trained on historical data to improve accuracy over time.
- Actionable Insights: The insights generated from the analysis can inform maintenance schedules, allowing technicians to address issues before they escalate into significant problems.
Implementing AI-Driven Predictive Maintenance
Successful implementation of AI-driven predictive maintenance requires a strategic approach. Telecom companies must invest in the right tools and technologies to harness the power of AI effectively.
Examples of AI Research Tools and Products
Several AI-driven products are available that can facilitate predictive maintenance in telecom networks:
- IBM Watson IoT: This platform integrates AI with IoT data to provide real-time insights into network performance. By using Watson’s machine learning capabilities, telecom operators can predict equipment failures and optimize maintenance schedules.
- Siemens MindSphere: An industrial IoT as a service solution, MindSphere utilizes advanced analytics and AI to monitor equipment health. Its predictive maintenance features help telecom companies reduce unplanned downtime and extend the lifecycle of network assets.
- GE Predix: Designed for industrial applications, Predix leverages AI to analyze data from network operations. Its predictive maintenance capabilities allow telecom providers to anticipate failures and reduce maintenance costs.
Case Studies in Telecom
Several telecom companies have successfully implemented AI-driven predictive maintenance, yielding impressive results.
Case Study 1: Vodafone
Vodafone adopted AI-driven analytics to monitor its network infrastructure. By analyzing historical data and real-time performance metrics, Vodafone was able to predict equipment failures with a 95% accuracy rate, significantly reducing downtime and improving customer satisfaction.
Case Study 2: AT&T
AT&T implemented a predictive maintenance system that utilizes machine learning algorithms to analyze network data. This initiative has led to a 30% reduction in service interruptions, demonstrating the effectiveness of AI in enhancing network reliability.
Future Trends in AI-Driven Predictive Maintenance
As AI technology continues to evolve, the potential for predictive maintenance in telecom networks will expand. Future trends may include:
- Increased Automation: Automation of maintenance tasks based on AI predictions will streamline operations and reduce human error.
- Enhanced Data Integration: Integrating data from diverse sources, including customer feedback and external environmental factors, will provide a more comprehensive view of network health.
- Real-Time Decision Making: Advancements in AI will enable real-time decision-making capabilities, allowing telecom companies to respond instantly to potential issues.
Conclusion
AI-driven predictive maintenance represents a transformative approach for the telecommunications industry, offering the potential to significantly reduce downtime and enhance network reliability. By investing in AI research tools and implementing effective strategies, telecom operators can not only improve operational efficiency but also foster greater customer loyalty. As the technology continues to advance, the future of predictive maintenance in telecom looks promising, paving the way for a more resilient and responsive network infrastructure.
Keyword: AI predictive maintenance telecom networks