AI Predictive Maintenance Reduces Downtime in Telecom Networks
Topic: AI Data Tools
Industry: Telecommunications
Discover how AI-powered predictive maintenance reduces downtime in telecom networks by leveraging data analytics to enhance efficiency and improve service quality
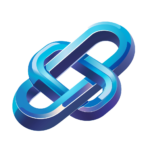
AI-Powered Predictive Maintenance: Reducing Downtime in Telecom Networks
Understanding Predictive Maintenance in Telecommunications
In the fast-paced world of telecommunications, maintaining network uptime is critical for service providers. Predictive maintenance, a proactive approach to equipment management, leverages data analytics and artificial intelligence (AI) to foresee potential failures before they occur. This strategy not only enhances operational efficiency but also significantly reduces downtime, ultimately improving customer satisfaction.
The Role of AI in Predictive Maintenance
Artificial intelligence plays a pivotal role in predictive maintenance by analyzing vast amounts of data generated by telecom networks. By employing machine learning algorithms, AI can identify patterns and anomalies that may indicate impending equipment failures. This capability allows telecom operators to perform maintenance tasks at optimal times, thus minimizing disruptions and extending the lifespan of their assets.
Key AI Techniques for Predictive Maintenance
- Machine Learning: Algorithms analyze historical data to predict when equipment is likely to fail.
- Natural Language Processing: Used to interpret unstructured data from maintenance logs and customer reports.
- Computer Vision: Inspects physical assets through image recognition to detect wear and tear.
Implementing AI-Powered Predictive Maintenance
To successfully implement AI-driven predictive maintenance, telecom companies must adopt a systematic approach. This includes data collection, model training, and integration of AI tools into existing workflows.
Step 1: Data Collection
The first step involves gathering data from various sources, including network devices, customer feedback, and historical maintenance records. This data serves as the foundation for AI algorithms to analyze and learn from.
Step 2: Model Training
Once sufficient data is collected, machine learning models can be trained to recognize patterns associated with equipment failures. This process requires collaboration between data scientists and domain experts to ensure the models are accurate and relevant.
Step 3: Integration of AI Tools
After training, the AI models must be integrated into the telecom company’s operational framework. This may involve deploying specific AI tools that facilitate real-time monitoring and alerts for maintenance needs.
Examples of AI-Driven Tools in Telecommunications
Several AI-powered tools and platforms can enhance predictive maintenance in telecom networks:
1. IBM Watson IoT
IBM Watson IoT offers advanced analytics capabilities that enable telecom operators to monitor equipment health in real-time. By analyzing sensor data, the platform can predict failures and recommend maintenance actions.
2. GE Digital’s Predix
Predix, developed by General Electric, is designed for industrial IoT applications. It provides predictive analytics that helps telecom companies optimize asset performance and reduce downtime through actionable insights.
3. Siemens MindSphere
Siemens MindSphere is an open cloud-based IoT operating system that allows telecom operators to connect their devices and analyze data. Its predictive maintenance capabilities help in forecasting equipment failures, thereby facilitating timely interventions.
Conclusion
The integration of AI-powered predictive maintenance in telecom networks is not merely an innovation; it is a necessity in today’s competitive landscape. By leveraging advanced AI tools and techniques, telecom operators can significantly reduce downtime, enhance operational efficiency, and improve overall service quality. As the industry continues to evolve, embracing AI-driven solutions will be crucial for staying ahead of the curve.
Keyword: AI predictive maintenance telecom networks