AI Privacy Solutions for Protecting Genomic Data in Bioeconomy
Topic: AI Privacy Tools
Industry: Pharmaceuticals and Biotechnology
Explore AI privacy solutions for genomic data protection in biotechnology Learn how innovative technologies enhance security and compliance in the bioeconomy
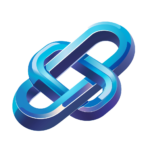
Securing the Bioeconomy: AI Privacy Solutions for Genomic Data Protection
Introduction to AI Privacy in Biotechnology
As the pharmaceutical and biotechnology sectors continue to evolve, the need for robust data protection mechanisms has never been more critical. The bioeconomy, which relies heavily on genomic data, faces unique challenges in safeguarding sensitive information. Artificial intelligence (AI) offers innovative solutions to enhance privacy and security, ensuring that genomic data is protected while enabling research and development.
The Importance of Genomic Data Protection
Genomic data is inherently sensitive and can reveal personal health information about individuals. This data is crucial for advancements in personalized medicine, drug development, and biotechnological innovations. However, the potential for misuse or unauthorized access to this information poses significant risks. Hence, implementing effective AI-driven privacy solutions is paramount for organizations operating in these fields.
AI-Driven Privacy Solutions
AI technologies can be harnessed to create sophisticated privacy solutions that not only protect genomic data but also comply with regulatory frameworks such as GDPR and HIPAA. Below are several key AI-driven tools and strategies that can be utilized:
1. Differential Privacy
Differential privacy is a mathematical framework that allows organizations to analyze and share data while ensuring that individuals’ privacy is maintained. By adding controlled noise to datasets, AI algorithms can generate insights without revealing specific information about individuals. Tools such as Google’s Differential Privacy library provide a robust foundation for implementing this technique in genomic studies.
2. Federated Learning
Federated learning allows AI models to be trained across decentralized devices holding local data without the need to transfer sensitive genomic information to a central server. This method ensures that data remains within its original environment, significantly reducing the risk of data breaches. Companies like NVIDIA and Intel are developing federated learning platforms tailored for healthcare applications, enabling secure collaboration among researchers.
3. AI-Powered Anonymization Tools
Anonymization is a critical step in protecting genomic data. AI-powered anonymization tools can automatically identify and redact personally identifiable information (PII) from datasets. For instance, tools like ARX Data Anonymization Tool use machine learning algorithms to enhance the anonymization process, ensuring that data can be used for research without compromising individual privacy.
4. Blockchain for Data Integrity
Blockchain technology offers a decentralized approach to data management, providing an immutable ledger that enhances the integrity and security of genomic data. AI can be integrated with blockchain to monitor access and usage patterns, ensuring compliance with privacy regulations. Companies like Chronicled are pioneering the use of blockchain in the pharmaceutical industry to secure supply chains and protect sensitive data.
5. Predictive Analytics for Threat Detection
AI-driven predictive analytics can help organizations identify potential security threats before they materialize. By analyzing patterns and anomalies in data access and usage, AI tools can alert stakeholders to suspicious activities. Platforms like Darktrace use machine learning to detect and respond to cyber threats in real-time, providing an additional layer of security for genomic data.
Case Studies in AI Privacy Implementation
Several organizations are already leveraging AI privacy solutions to protect genomic data:
Case Study 1: Genomics England
Genomics England has implemented differential privacy techniques to analyze genomic data while safeguarding patient identities. By using AI to anonymize datasets, the organization can share valuable insights with researchers without compromising individual privacy.
Case Study 2: 23andMe
23andMe employs federated learning to enhance its genomic analysis capabilities. By training AI models on user data without transferring sensitive information, the company can ensure user privacy while still deriving valuable insights for research and product development.
Conclusion
As the bioeconomy continues to expand, the protection of genomic data must remain a top priority. AI-driven privacy solutions offer powerful tools for the pharmaceutical and biotechnology sectors to secure sensitive information while fostering innovation. By adopting these technologies, organizations can not only comply with regulatory requirements but also build trust with stakeholders, ultimately contributing to the advancement of healthcare and biotechnology.
Keyword: AI privacy solutions for genomic data