AI Privacy Tools for Secure Collaborative Manufacturing
Topic: AI Privacy Tools
Industry: Manufacturing
Discover how AI privacy tools enhance data security in collaborative manufacturing and safeguard sensitive information while boosting operational efficiency.
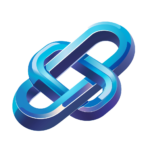
Securing the Supply Chain: AI Privacy Tools for Collaborative Manufacturing
Introduction to AI in Manufacturing
The manufacturing sector is undergoing a significant transformation through the adoption of artificial intelligence (AI). As companies increasingly collaborate across supply chains, the need for robust privacy measures becomes paramount. AI privacy tools are designed to safeguard sensitive data while enhancing operational efficiency. This article explores how AI can be effectively implemented in collaborative manufacturing environments and highlights specific tools that can bolster supply chain security.
The Importance of Data Privacy in Manufacturing
In an interconnected manufacturing landscape, data privacy is not just a regulatory requirement but a critical component of trust and reliability. Manufacturers often share sensitive information with partners, including product designs, production schedules, and customer data. A breach in this data can lead to significant financial losses, reputational damage, and legal ramifications. Therefore, implementing AI privacy tools is essential for protecting this information while maintaining collaborative efforts.
AI Privacy Tools: An Overview
AI privacy tools utilize advanced algorithms and machine learning techniques to analyze, encrypt, and manage data securely. These tools can help manufacturers mitigate risks associated with data sharing and ensure compliance with various privacy regulations. The following sections will explore specific AI-driven products that can enhance privacy in collaborative manufacturing.
1. Differential Privacy
Differential privacy is a technique that allows organizations to extract insights from datasets without compromising individual data points. By adding noise to the data, manufacturers can share aggregated information with partners while keeping sensitive details confidential. Tools like Google’s Differential Privacy library provide frameworks for implementing this technology, enabling manufacturers to analyze trends without exposing proprietary information.
2. Federated Learning
Federated learning is an AI approach that allows multiple parties to collaborate on model training without sharing their raw data. This method is particularly useful in manufacturing, where companies can improve predictive maintenance models while keeping their operational data secure. Platforms such as TensorFlow Federated enable manufacturers to develop machine learning models collaboratively, ensuring data privacy throughout the process.
3. Blockchain Technology
Blockchain offers a decentralized approach to data management that enhances transparency and security within supply chains. By recording transactions on a tamper-proof ledger, manufacturers can track the provenance of materials and ensure compliance with regulations. AI-driven blockchain solutions, such as IBM’s Food Trust, leverage artificial intelligence to analyze data across the supply chain while maintaining privacy and security.
4. AI-Powered Access Control
Implementing robust access control measures is vital for safeguarding sensitive data. AI-powered access control systems can analyze user behavior and patterns to detect anomalies, thereby preventing unauthorized access. Tools like Okta utilize machine learning to provide adaptive authentication and identity management solutions, ensuring that only authorized personnel can access critical information.
Case Studies: Successful Implementation of AI Privacy Tools
Case Study 1: Siemens
Siemens has successfully integrated AI-driven privacy tools into its manufacturing processes. By utilizing differential privacy techniques, the company can share insights with supply chain partners while protecting sensitive design data. This approach has not only enhanced collaboration but also ensured compliance with data protection regulations.
Case Study 2: General Motors
General Motors has adopted federated learning to improve its predictive maintenance capabilities. By allowing different manufacturing plants to train machine learning models without sharing their raw data, GM has enhanced its operational efficiency while maintaining strict data privacy standards. This innovative approach has led to reduced downtime and increased productivity.
Conclusion
As the manufacturing industry continues to evolve, the integration of AI privacy tools is becoming increasingly essential for securing the supply chain. By leveraging techniques such as differential privacy, federated learning, blockchain technology, and AI-powered access control, manufacturers can protect sensitive data while fostering collaboration. The successful implementation of these tools not only enhances data security but also drives innovation and efficiency across the supply chain.
Keyword: AI privacy tools for manufacturing