AI Quality Control in Manufacturing Defect Detection Systems
Topic: AI Design Tools
Industry: Manufacturing
Discover how AI-powered quality control enhances defect detection in manufacturing with real-time monitoring increased accuracy and cost efficiency
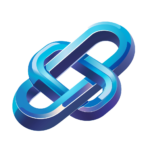
AI-Powered Quality Control: Designing Defect Detection Systems
Introduction to AI in Manufacturing
In the ever-evolving landscape of manufacturing, quality control remains a critical component for ensuring product excellence. As the industry embraces technological advancements, artificial intelligence (AI) has emerged as a game-changer, particularly in the realm of defect detection systems. This article delves into the implementation of AI in quality control, highlighting specific tools and products that can enhance defect detection processes.
The Role of AI in Quality Control
AI technologies, such as machine learning and computer vision, are revolutionizing quality control by automating the detection of defects in manufacturing processes. These technologies can analyze vast amounts of data at unprecedented speeds, identifying patterns and anomalies that may go unnoticed by human inspectors. The result is a more efficient, accurate, and scalable quality control process.
Key Benefits of AI-Powered Quality Control
- Increased Accuracy: AI systems can achieve higher accuracy rates in defect detection compared to traditional methods, reducing false positives and negatives.
- Real-Time Monitoring: AI-driven tools can provide continuous monitoring of production lines, allowing for immediate corrective actions to be taken when defects are detected.
- Cost Efficiency: By minimizing waste and rework, AI-powered systems can significantly lower operational costs associated with quality control.
- Data-Driven Insights: AI can analyze historical data to predict potential defects, enabling proactive measures to improve manufacturing processes.
Implementing AI in Defect Detection Systems
To successfully integrate AI into defect detection systems, manufacturers should consider the following steps:
1. Identifying Use Cases
Manufacturers must first identify specific use cases where AI can add value. Common applications include visual inspection of products, monitoring assembly lines, and analyzing sensor data for quality assurance.
2. Selecting the Right Tools
Choosing the appropriate AI tools is crucial for effective implementation. Several AI-driven products have gained traction in the manufacturing sector:
Vision AI
Tools such as Google Cloud Vision AI enable manufacturers to automate visual inspections. By training the model with images of both defective and non-defective products, the system can learn to identify defects in real-time.
Machine Learning Platforms
Platforms like IBM Watson Studio allow manufacturers to develop custom machine learning models tailored to their specific quality control needs. These models can analyze historical data to predict defects and optimize processes.
IoT Integration
Integrating AI with the Internet of Things (IoT) can enhance defect detection capabilities. For instance, Siemens MindSphere utilizes IoT data to monitor equipment performance and detect anomalies that may indicate quality issues.
3. Training and Adoption
Once the tools are selected, it is essential to train staff on how to use these AI systems effectively. This includes understanding how to interpret AI-generated insights and make data-driven decisions.
Case Studies of Successful Implementation
Several companies have successfully implemented AI-powered defect detection systems, yielding impressive results:
Case Study: Tesla
Tesla has incorporated AI-driven visual inspection systems in its manufacturing plants. By utilizing advanced computer vision techniques, Tesla has significantly reduced the time required for quality inspections, leading to faster production cycles and improved product quality.
Case Study: Bosch
Bosch employs AI algorithms to analyze sensor data from its manufacturing equipment. This proactive approach allows the company to predict potential defects before they occur, ensuring higher quality standards and minimizing downtime.
Conclusion
The integration of AI in quality control is not merely a trend but a necessity for manufacturers aiming to maintain competitiveness in a rapidly changing market. By designing effective defect detection systems powered by AI, companies can enhance their quality assurance processes, reduce costs, and ultimately deliver superior products to their customers. As technology continues to advance, the potential for AI in manufacturing will only grow, paving the way for a more efficient and reliable industry.
Keyword: AI powered quality control systems