AI Quality Control in Pharmaceutical Manufacturing Revolution
Topic: AI Data Tools
Industry: Pharmaceuticals
Discover how AI-powered quality control is transforming pharmaceutical manufacturing by enhancing accuracy reducing costs and ensuring compliance for better patient outcomes
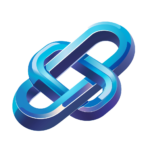
AI-Powered Quality Control: Transforming Pharmaceutical Manufacturing
Introduction to AI in Pharmaceutical Manufacturing
The pharmaceutical industry is under constant pressure to enhance product quality, ensure compliance with regulatory standards, and reduce operational costs. In this landscape, artificial intelligence (AI) is emerging as a transformative force, particularly in the realm of quality control. By leveraging advanced AI data tools, pharmaceutical manufacturers can significantly improve their quality assurance processes, leading to more efficient operations and better patient outcomes.
Understanding AI-Powered Quality Control
AI-powered quality control involves the use of machine learning algorithms, predictive analytics, and data processing capabilities to monitor and enhance the quality of pharmaceutical products. These technologies can analyze vast amounts of data in real-time, identifying anomalies and trends that may indicate potential quality issues. This proactive approach not only minimizes risks but also streamlines the quality control workflow.
Key Benefits of AI in Quality Control
- Enhanced Accuracy: AI algorithms can detect subtle variations in product quality that may be overlooked by human inspectors.
- Predictive Maintenance: By analyzing historical data, AI can predict equipment failures before they occur, reducing downtime.
- Real-Time Monitoring: Continuous data analysis allows for immediate identification of quality deviations, enabling swift corrective actions.
- Cost Reduction: Automating quality control processes can lead to significant savings on labor and operational expenses.
Implementing AI in Quality Control
To successfully implement AI in quality control, pharmaceutical companies must adopt a strategic approach that includes the following steps:
1. Data Collection and Integration
Effective AI solutions rely on high-quality data. Pharmaceutical manufacturers should invest in robust data collection systems that integrate various sources of information, including production data, laboratory results, and supplier information. This data serves as the foundation for AI algorithms to learn and make informed decisions.
2. Selecting the Right AI Tools
Choosing the appropriate AI tools is crucial for successful implementation. Several AI-driven products have gained traction in the pharmaceutical sector:
Machine Learning Platforms
Tools like IBM Watson and Google Cloud AI provide machine learning capabilities that can be tailored to specific quality control needs. These platforms enable companies to develop custom models that analyze production data and identify quality trends.
Vision Inspection Systems
AI-powered vision systems, such as Cognex and Keyence, utilize computer vision technology to inspect products for defects. These systems can operate at high speeds and with greater accuracy than traditional inspection methods, ensuring that only products meeting quality standards reach the market.
Predictive Analytics Tools
Solutions like SAS Analytics and Tableau offer predictive analytics capabilities that can forecast potential quality issues based on historical data. By identifying patterns and trends, these tools help manufacturers take proactive measures to mitigate risks.
3. Training and Change Management
Implementing AI technology requires a cultural shift within the organization. Employees must be trained to understand and utilize AI tools effectively. Change management strategies should be employed to ensure a smooth transition and to foster a mindset of continuous improvement.
Case Studies: AI in Action
Several pharmaceutical companies have already begun to reap the benefits of AI-powered quality control:
Case Study 1: Pfizer
Pfizer has implemented AI-driven analytics to monitor production processes in real-time. By utilizing machine learning algorithms, they can predict potential quality issues and adjust their processes accordingly, resulting in a significant reduction in product recalls.
Case Study 2: Novartis
Novartis has adopted AI-powered vision inspection systems to enhance their quality control measures. These systems have improved defect detection rates and have streamlined the inspection process, leading to faster time-to-market for new products.
Conclusion
AI-powered quality control is revolutionizing the pharmaceutical manufacturing landscape. By implementing advanced AI tools and strategies, companies can enhance product quality, reduce operational costs, and ensure compliance with regulatory standards. As the industry continues to evolve, those who embrace AI will be well-positioned to lead in quality assurance and overall operational excellence.
Keyword: AI quality control in pharmaceuticals