AI Revolutionizing Drug Discovery and Reducing Pharma Costs
Topic: AI Finance Tools
Industry: Pharmaceuticals
Discover how AI is transforming drug discovery in pharma by reducing R&D costs and accelerating the development of new therapies for better patient outcomes
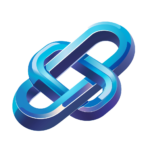
How AI is Revolutionizing Drug Discovery and Cutting R&D Costs in Pharma
The Role of AI in Pharmaceutical Research
Artificial intelligence (AI) is transforming the pharmaceutical industry by streamlining drug discovery processes and significantly reducing research and development (R&D) costs. Traditional drug discovery is often a lengthy and expensive endeavor, typically taking over a decade and costing billions of dollars. However, AI-driven tools are reshaping this landscape, enabling pharmaceutical companies to enhance efficiency and make data-driven decisions.
AI Implementation in Drug Discovery
Implementing AI in drug discovery involves integrating advanced algorithms and machine learning techniques into various stages of the research process. These technologies can analyze vast datasets, predict molecular behavior, and identify potential drug candidates more quickly than traditional methods.
Data Analysis and Predictive Modeling
AI excels at processing large volumes of data, which is crucial in drug discovery. Machine learning algorithms can sift through genomic data, clinical trial results, and existing pharmaceutical research to identify patterns and correlations that may not be immediately apparent to human researchers. For instance, tools like IBM Watson for Drug Discovery utilize natural language processing and machine learning to uncover hidden relationships in data, accelerating the identification of viable drug candidates.
Virtual Screening and Compound Optimization
AI can significantly enhance the virtual screening process, where thousands of compounds are evaluated for their potential effectiveness. Tools such as Schrödinger and Atomwise leverage AI to predict how different compounds will interact with specific biological targets. By using deep learning algorithms, these platforms can optimize molecular structures, reducing the time and resources required for laboratory testing.
Examples of AI-Driven Products in Pharma
Several AI-driven products are already making waves in the pharmaceutical sector, showcasing the potential of this technology to revolutionize drug discovery.
Insilico Medicine
Insilico Medicine is a notable player in the field, utilizing AI to accelerate drug discovery and development. Their platform combines machine learning with generative adversarial networks (GANs) to design novel drug candidates and predict their effectiveness, thereby shortening the drug development timeline.
BenevolentAI
BenevolentAI employs AI to analyze scientific literature and clinical data to identify new therapeutic targets and drug candidates. Their platform has successfully identified promising drug candidates for various diseases, demonstrating the power of AI in translating data into actionable insights.
Deep Genomics
Deep Genomics focuses on using AI to predict the effects of genetic mutations on drug responses. Their technology enables pharmaceutical companies to tailor treatments to individual patients, thereby improving outcomes and reducing costs associated with trial and error in drug prescriptions.
Cost Reduction through AI Integration
The integration of AI into drug discovery processes can lead to substantial cost savings for pharmaceutical companies. By streamlining R&D processes, reducing the number of failed clinical trials, and optimizing resource allocation, AI can help companies bring drugs to market faster and at a lower cost.
Reducing Clinical Trial Failures
One of the major contributors to high R&D costs is the high rate of clinical trial failures. AI can enhance patient selection for trials, ensuring that the right candidates are chosen based on genetic and phenotypic data. This targeted approach can lead to a higher success rate in clinical trials, ultimately reducing costs.
Accelerating Time to Market
AI tools can also expedite the drug development timeline. By automating repetitive tasks and providing predictive analytics, researchers can focus on high-value activities, leading to faster innovation cycles and quicker market entry for new therapies.
Conclusion
As the pharmaceutical industry continues to embrace AI technologies, the potential for innovation and cost reduction in drug discovery is immense. By leveraging AI-driven tools and platforms, pharmaceutical companies can enhance their research capabilities, reduce R&D costs, and ultimately bring life-saving drugs to market more efficiently. The future of drug discovery lies in the intelligent integration of AI, paving the way for a new era in pharmaceutical research and development.
Keyword: AI in drug discovery