AI Revolutionizing Drug Discovery Trends and Tools in 2025
Topic: AI Data Tools
Industry: Pharmaceuticals
Discover how AI is transforming drug discovery in 2025 with innovative tools and trends enhancing efficiency and accelerating new therapies development
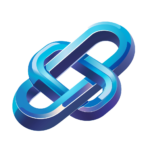
How AI is Revolutionizing Drug Discovery in 2025: Key Tools and Trends
Introduction to AI in Drug Discovery
As we progress through 2025, the pharmaceutical industry is witnessing a paradigm shift driven by artificial intelligence (AI). The integration of AI data tools is transforming drug discovery processes, enhancing efficiency, and accelerating the development of new therapies. This article explores the key tools and trends in AI that are reshaping the landscape of drug discovery.
AI-Powered Drug Discovery Platforms
One of the most significant advancements in drug discovery is the emergence of AI-powered platforms that streamline various stages of the research process. These platforms utilize machine learning algorithms to analyze vast datasets, predict molecular behavior, and identify potential drug candidates.
1. Atomwise
Atomwise is a leading AI-driven platform that employs deep learning to predict the binding affinity of small molecules to proteins. By leveraging a proprietary database of known compounds, Atomwise can rapidly screen millions of potential drug candidates, significantly reducing the time and cost associated with traditional drug discovery methods.
2. BenevolentAI
BenevolentAI focuses on harnessing AI to uncover new drug targets and repurpose existing drugs. Their platform integrates various data sources, including scientific literature and clinical trial data, to generate insights that guide researchers in identifying novel therapeutic approaches.
Machine Learning Algorithms in Drug Design
Machine learning algorithms are at the forefront of AI applications in drug design. These algorithms can analyze complex biological data, enabling researchers to make informed decisions during the drug development process.
1. Generative Adversarial Networks (GANs)
GANs are being utilized to generate new molecular structures that meet specific criteria. By training on existing drug compounds, GANs can create novel candidates that have the potential to be effective therapeutics. This approach not only accelerates the discovery process but also expands the chemical space that researchers can explore.
2. Reinforcement Learning
Reinforcement learning is another powerful tool in drug discovery. It allows AI systems to learn from trial and error, optimizing the drug design process by predicting the outcomes of various chemical modifications. This iterative approach enhances the likelihood of identifying successful drug candidates.
Data Integration and Analysis Tools
The successful implementation of AI in drug discovery relies heavily on robust data integration and analysis tools. These tools enable researchers to consolidate diverse datasets, facilitating comprehensive analyses that inform decision-making.
1. IBM Watson for Drug Discovery
IBM Watson for Drug Discovery is a prime example of a data integration tool that leverages AI to assist researchers in identifying potential drug candidates. By analyzing scientific literature, clinical trial data, and genomic information, Watson provides insights that can lead to the discovery of new therapies.
2. BioSymphony
BioSymphony is an AI-driven platform that focuses on data harmonization and analysis in the pharmaceutical industry. By integrating data from various sources, BioSymphony enables researchers to gain a holistic view of drug discovery processes, improving collaboration and accelerating timelines.
Trends Shaping the Future of AI in Drug Discovery
As AI continues to evolve, several trends are emerging that will further influence drug discovery in the coming years.
1. Increased Collaboration Between AI and Traditional Research
The pharmaceutical industry is witnessing a growing collaboration between AI technology providers and traditional research institutions. This partnership enhances the validation of AI-driven insights and fosters a culture of innovation.
2. Regulatory Acceptance of AI Solutions
Regulatory bodies are increasingly recognizing the potential of AI in drug discovery. As guidelines evolve, the acceptance of AI-driven solutions will facilitate faster approvals and integration into clinical workflows.
3. Focus on Personalized Medicine
The integration of AI tools is paving the way for personalized medicine. By analyzing patient data, AI can help identify specific treatments tailored to individual genetic profiles, improving treatment efficacy and patient outcomes.
Conclusion
The revolution brought about by AI in drug discovery is undeniable. As we move further into 2025, the adoption of AI data tools will continue to reshape the pharmaceutical landscape, driving innovation and efficiency in the quest for new therapies. By embracing these technologies, the industry can look forward to a future where drug discovery is faster, more accurate, and ultimately more successful.
Keyword: AI in drug discovery 2025