AI Search Tools Transform Predictive Maintenance in Energy Sector
Topic: AI Search Tools
Industry: Energy and Utilities
Discover how AI search tools are revolutionizing predictive maintenance in the energy sector enhancing reliability reducing costs and optimizing power grid operations
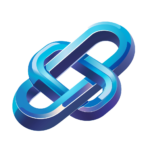
Predictive Maintenance Reimagined: AI Search Tools for Power Grid Optimization
Understanding Predictive Maintenance in the Energy Sector
Predictive maintenance has emerged as a critical strategy for optimizing operations within the energy and utilities sector. By leveraging advanced data analytics, organizations can forecast equipment failures before they occur, thereby minimizing downtime and reducing maintenance costs. The integration of artificial intelligence (AI) into predictive maintenance processes further enhances these capabilities, allowing for real-time insights and improved decision-making.
The Role of AI Search Tools
AI search tools have revolutionized how energy companies analyze and interpret vast amounts of data generated by power grids. These tools utilize machine learning algorithms to sift through historical data, identifying patterns and anomalies that may indicate potential failures. The shift from traditional maintenance strategies to AI-driven predictive maintenance is not just a trend; it is a necessity for companies seeking to stay competitive in a rapidly evolving market.
Key Benefits of AI-Driven Predictive Maintenance
- Increased Reliability: By predicting when equipment is likely to fail, companies can schedule maintenance at optimal times, increasing the reliability of power supply.
- Cost Reduction: Early detection of issues can lead to significant savings by preventing catastrophic failures that require expensive repairs.
- Enhanced Efficiency: AI tools enable utilities to optimize their maintenance schedules, ensuring that resources are allocated effectively.
Implementing AI Search Tools in Predictive Maintenance
To effectively implement AI search tools for predictive maintenance in power grids, organizations must consider several factors, including data integration, tool selection, and workforce training.
Data Integration
Successful implementation begins with the seamless integration of data from various sources, including sensors, SCADA systems, and historical maintenance records. This data serves as the foundation for AI algorithms to analyze and generate actionable insights.
Choosing the Right Tools
Several AI-driven products are available that can enhance predictive maintenance efforts within the energy sector:
- IBM Maximo: This asset management solution utilizes AI to predict equipment failures and optimize maintenance schedules, allowing utilities to maintain operational efficiency.
- GE Digital’s Predix: Predix combines data analytics and machine learning to provide insights into asset performance, enabling predictive maintenance strategies tailored to specific operational needs.
- Siemens MindSphere: As an industrial IoT platform, MindSphere connects various devices and systems, offering advanced analytics capabilities that support predictive maintenance initiatives.
Workforce Training
To fully harness the potential of AI search tools, it is crucial to invest in training for personnel. Employees must understand how to interpret AI-generated insights and apply them to their maintenance strategies. This training ensures that the workforce is equipped to make data-driven decisions that align with organizational goals.
Case Studies: Successful Implementation of AI Search Tools
Several energy companies have successfully implemented AI-driven predictive maintenance strategies, showcasing the benefits of these advanced technologies:
Case Study 1: Duke Energy
Duke Energy has integrated AI analytics into its predictive maintenance framework, significantly reducing unplanned outages. By analyzing data from thousands of sensors across its grid, the company has improved its ability to predict equipment failures, leading to enhanced service reliability for its customers.
Case Study 2: Enel
Enel, a global energy company, utilizes AI search tools to optimize its maintenance processes. By employing machine learning algorithms, Enel has been able to predict failures in wind turbines and other assets, resulting in reduced maintenance costs and increased operational efficiency.
Conclusion
The integration of AI search tools into predictive maintenance strategies is transforming the energy and utilities sector. By harnessing the power of artificial intelligence, companies can optimize their operations, reduce costs, and enhance the reliability of their power grids. As the industry continues to evolve, those who embrace these innovative technologies will undoubtedly lead the way in operational excellence.
Keyword: AI predictive maintenance tools