AI Self Learning Algorithms Boost Energy Efficiency in Power Plants
Topic: AI Self Improvement Tools
Industry: Energy and Utilities
Discover how AI self-learning algorithms enhance energy efficiency in power plants by optimizing operations reducing costs and supporting sustainability initiatives
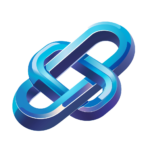
AI Self-Learning Algorithms: Enhancing Energy Efficiency in Power Plants
Understanding AI Self-Learning Algorithms
Artificial intelligence (AI) has revolutionized various industries, and the energy sector is no exception. Self-learning algorithms, a subset of AI, enable systems to improve their performance over time by analyzing data and identifying patterns without explicit programming. These algorithms are particularly beneficial in power plants, where operational efficiency and energy management are crucial for sustainability and cost-effectiveness.
Implementing AI in Power Plants
Integrating AI self-learning algorithms into power plant operations involves several key steps:
1. Data Collection and Analysis
The first step is to gather extensive data from various sources within the power plant, including sensors, meters, and control systems. This data serves as the foundation for training AI models. Advanced analytics tools can be employed to process this data, identifying trends and anomalies that may not be immediately apparent.
2. Algorithm Development
Once the data is collected, self-learning algorithms can be developed. These algorithms utilize machine learning techniques, such as supervised and unsupervised learning, to create models that can predict outcomes based on historical data. For example, predictive maintenance algorithms can forecast equipment failures, allowing for timely interventions that prevent costly downtimes.
3. Continuous Improvement
AI self-learning algorithms continuously refine their predictions and recommendations as new data becomes available. This iterative process ensures that the algorithms remain relevant and effective, adapting to changes in operational conditions and energy demand.
Examples of AI-Driven Tools in Energy Management
Several AI-driven products and tools are currently enhancing energy efficiency in power plants:
1. Siemens’ MindSphere
Siemens’ MindSphere is an industrial IoT platform that leverages AI to optimize energy management. By connecting machines and systems, MindSphere collects real-time data that can be analyzed to improve operational efficiency. The platform’s self-learning capabilities allow it to identify patterns and suggest optimizations, reducing energy consumption and operational costs.
2. GE Digital’s Predix
GE Digital’s Predix platform offers advanced analytics and machine learning capabilities tailored for the energy sector. Predix enables power plants to monitor equipment performance, predict maintenance needs, and optimize energy production. The platform’s self-learning algorithms analyze historical and real-time data, providing actionable insights that enhance overall efficiency.
3. IBM Watson for Energy
IBM Watson for Energy uses AI to drive insights from vast amounts of operational data. The platform supports predictive maintenance, energy forecasting, and operational optimization. By employing self-learning algorithms, Watson can identify inefficiencies and recommend strategies to improve energy usage, ultimately leading to reduced operational costs and a smaller carbon footprint.
Benefits of AI Self-Learning Algorithms in Power Plants
The implementation of AI self-learning algorithms in power plants offers numerous advantages:
1. Improved Operational Efficiency
By predicting equipment failures and optimizing maintenance schedules, power plants can reduce downtime and enhance productivity.
2. Enhanced Energy Management
AI algorithms can analyze energy consumption patterns, leading to more efficient energy use and reduced waste.
3. Cost Savings
With improved efficiency and reduced downtime, power plants can achieve significant cost savings, allowing for reinvestment in sustainable technologies.
4. Environmental Impact
By optimizing energy usage and reducing emissions, AI self-learning algorithms contribute to a more sustainable energy future.
Conclusion
AI self-learning algorithms represent a transformative force in the energy sector, particularly in power plants. By harnessing the power of data and advanced analytics, these algorithms enhance energy efficiency, reduce operational costs, and support sustainability initiatives. As technology continues to evolve, the integration of AI in energy management will undoubtedly play a pivotal role in shaping the future of power generation.
Keyword: AI self-learning algorithms energy efficiency