AI Solutions for Efficient Data Center Energy Management
Topic: AI Business Tools
Industry: Energy and Utilities
Discover how AI solutions can optimize energy management in data centers addressing rising energy demands through predictive maintenance and intelligent systems
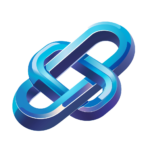
AI Solutions for Managing the Surge in Data Center Energy Demand
As the digital landscape continues to evolve, data centers are experiencing an unprecedented surge in energy demand. The proliferation of cloud computing, big data analytics, and the Internet of Things (IoT) has led to a significant increase in operational requirements. To address this challenge, artificial intelligence (AI) is emerging as a powerful tool for optimizing energy consumption and enhancing operational efficiency in data centers.
The Role of AI in Energy Management
AI technologies can be implemented across various facets of energy management within data centers. By leveraging machine learning algorithms and predictive analytics, organizations can gain valuable insights into their energy usage patterns, enabling them to make data-driven decisions aimed at reducing consumption and costs.
1. Predictive Maintenance
One of the primary applications of AI in data centers is predictive maintenance. By utilizing AI-driven tools such as IBM’s Watson IoT, organizations can monitor equipment health in real-time. This technology analyzes historical data and identifies patterns that indicate potential failures, allowing for timely interventions before issues arise. This not only minimizes downtime but also optimizes energy usage by ensuring that equipment operates efficiently.
2. Dynamic Energy Management
AI can also enhance dynamic energy management systems. Tools like Siemens’ EnergyIP leverage AI algorithms to analyze energy consumption data and provide recommendations for optimizing energy usage. These systems can automatically adjust power distribution based on real-time demand, ensuring that energy is utilized efficiently while minimizing waste.
3. Intelligent Cooling Systems
Cooling systems are one of the largest energy consumers in data centers. AI-driven solutions, such as those offered by Schneider Electric, utilize machine learning to optimize cooling operations. By analyzing temperature and humidity data, these systems can adjust cooling output dynamically, reducing energy consumption while maintaining optimal operating conditions for servers.
AI-Driven Analytics Platforms
In addition to specific applications, AI-driven analytics platforms offer comprehensive solutions for energy management. Tools like Daintree Networks provide organizations with a holistic view of their energy consumption patterns. By integrating AI with IoT devices, these platforms can identify inefficiencies and recommend strategies for improvement, ultimately leading to significant energy savings.
4. Demand Response Programs
AI can also facilitate participation in demand response programs, where data centers can reduce or shift their energy usage during peak demand periods. Solutions like Enel X’s demand response platform utilize AI to predict peak times and automate responses, allowing data centers to contribute to grid stability while benefiting from financial incentives.
Conclusion
As data centers grapple with increasing energy demands, the integration of AI solutions presents a viable path toward sustainable energy management. By adopting AI-driven tools for predictive maintenance, dynamic energy management, intelligent cooling systems, and comprehensive analytics, organizations can not only optimize their energy consumption but also reduce operational costs. The future of energy management in data centers lies in leveraging the capabilities of artificial intelligence to create smarter, more efficient operations.
Keyword: AI energy management solutions