AI Strategies for Success in Quantitative Trading
Topic: AI Finance Tools
Industry: Investment Management
Discover how AI is transforming quantitative trading with strategies for data analysis predictive analytics and risk management to outperform the market
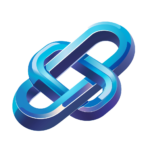
The AI Edge in Quantitative Trading: Strategies for Outperforming the Market
Understanding AI in Quantitative Trading
Artificial Intelligence (AI) is revolutionizing the landscape of quantitative trading by enabling investment managers to analyze vast amounts of data with unprecedented speed and accuracy. The integration of AI finance tools allows firms to identify patterns and trends that may not be visible through traditional analytical methods. This blog explores various strategies and tools that leverage AI to enhance investment decision-making and improve market performance.
Key Strategies for Implementing AI in Quantitative Trading
1. Data Collection and Preprocessing
Effective quantitative trading begins with robust data collection. AI-driven tools can automate the aggregation of diverse data sources, including historical market data, news sentiment, and social media trends. Tools like Alpaca and Quandl provide APIs to access a wealth of financial data, which can be preprocessed using machine learning algorithms to clean and structure the data for analysis.
2. Predictive Analytics
Once the data is prepared, predictive analytics come into play. AI algorithms such as neural networks and decision trees can be employed to forecast market movements. For instance, TensorFlow and PyTorch are popular frameworks that allow quantitative analysts to build models capable of predicting stock prices based on historical data and market indicators.
3. Algorithmic Trading
AI can enhance algorithmic trading strategies by optimizing trade execution and minimizing costs. Platforms like MetaTrader 5 offer AI-powered trading bots that can execute trades based on predefined criteria. These bots can adapt to changing market conditions in real-time, ensuring that trades are executed at the best possible prices.
4. Risk Management
Effective risk management is crucial in quantitative trading. AI tools can analyze risk factors and provide insights into potential market volatility. RiskMetrics and Palantir are examples of AI-driven risk management platforms that can help investment managers quantify risks and make informed decisions to mitigate them.
5. Continuous Learning and Adaptation
One of the most significant advantages of AI is its ability to learn from new data. Machine learning models can continuously improve their predictions by adapting to new market conditions. Tools like DataRobot and H2O.ai facilitate the development of adaptive algorithms that evolve as they are exposed to more data, ensuring that strategies remain relevant over time.
Examples of AI-Driven Products in Quantitative Trading
1. QuantConnect
QuantConnect is an open-source algorithmic trading platform that allows users to build, backtest, and deploy trading strategies using AI. Its cloud-based infrastructure supports various programming languages, making it accessible for both novice and experienced traders.
2. Trade Ideas
Trade Ideas utilizes AI to generate trade ideas and alerts based on real-time market data. Its AI engine, Holly, analyzes historical data to identify high-probability trading opportunities, allowing users to capitalize on market inefficiencies.
3. Bloomberg Terminal
The Bloomberg Terminal integrates AI capabilities to enhance financial analysis and trading decisions. Its advanced analytics tools provide insights into market trends and allow users to execute trades efficiently, making it an essential tool for investment managers.
Conclusion
As the financial landscape continues to evolve, the integration of AI in quantitative trading presents a significant opportunity for investment managers to outperform the market. By leveraging AI finance tools for data collection, predictive analytics, algorithmic trading, risk management, and continuous learning, firms can enhance their trading strategies and achieve superior returns. Embracing these technologies is no longer optional; it is essential for those looking to maintain a competitive edge in the fast-paced world of finance.
Keyword: AI in quantitative trading strategies