AI Summarization Tools for Effective Insurance Fraud Detection
Topic: AI Summarizer Tools
Industry: Insurance
Discover how AI summarization tools enhance insurance fraud detection by analyzing data patterns and improving operational efficiency in the fight against fraud
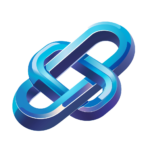
AI Summarization Tools: The Secret Weapon for Insurance Fraud Detection
Understanding the Role of AI in Insurance Fraud Detection
Insurance fraud remains a significant challenge for the industry, costing billions of dollars annually. As fraudulent activities become increasingly sophisticated, traditional methods of detection are often insufficient. This is where artificial intelligence (AI) comes into play. AI summarization tools can analyze vast amounts of data quickly and efficiently, allowing insurers to identify suspicious patterns and behaviors that may indicate fraud.
How AI Summarization Tools Work
AI summarization tools utilize natural language processing (NLP) and machine learning algorithms to extract relevant information from large datasets. These tools can process unstructured data, such as claim reports, customer communications, and social media interactions, summarizing key points and highlighting anomalies.
Key Features of AI Summarization Tools
- Data Extraction: Automatically identifies and extracts pertinent information from diverse data sources.
- Pattern Recognition: Uses machine learning to detect patterns that may suggest fraudulent activity.
- Real-Time Analysis: Provides immediate insights, allowing insurers to respond swiftly to potential fraud cases.
Implementing AI Summarization Tools in Insurance
For insurers looking to integrate AI summarization tools into their fraud detection processes, several steps can be taken:
1. Identify Data Sources
Begin by identifying the various data sources that can be utilized in fraud detection, including claims data, customer interactions, and external databases. The more comprehensive the data, the more effective the AI tools will be.
2. Choose the Right Tools
There are numerous AI-driven products available that can assist in summarizing and analyzing data for fraud detection. Some notable examples include:
- IBM Watson: Known for its advanced NLP capabilities, IBM Watson can analyze claims data and identify inconsistencies that may indicate fraud.
- Google Cloud Natural Language: This tool can extract insights from unstructured text, helping insurers summarize claims and detect anomalies.
- Lexalytics: Lexalytics offers sentiment analysis and text summarization features, enabling insurers to gauge customer sentiment and identify potential fraud indicators.
3. Train the AI Model
Training the AI model with historical fraud cases will enhance its ability to recognize fraudulent patterns. By feeding the model a diverse dataset, insurers can improve its accuracy and effectiveness over time.
4. Monitor and Adjust
Implementing AI tools is not a one-time effort. Continuous monitoring and adjustment are essential to ensure the tools remain effective as fraud tactics evolve. Regularly reviewing the AI’s performance and making necessary adjustments will help maintain its relevance and accuracy.
Conclusion: The Future of Fraud Detection in Insurance
AI summarization tools represent a significant advancement in the fight against insurance fraud. By leveraging these technologies, insurers can enhance their detection capabilities, reduce losses, and improve operational efficiency. As the industry continues to embrace AI, those who adopt these tools will be better positioned to combat fraud and protect their bottom line.
Keyword: AI tools for insurance fraud detection