AI Tools for Data Analysis and Visualization in Research
Topic: AI Writing Tools
Industry: Research and Academia
Discover how AI tools enhance data analysis and visualization in academic research empowering scholars to uncover insights and present findings effectively
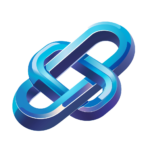
AI Tools for Data Analysis and Visualization in Academic Research
Introduction to AI in Academic Research
The integration of artificial intelligence (AI) into academic research has revolutionized the way scholars approach data analysis and visualization. By leveraging AI tools, researchers can enhance their ability to process vast amounts of data, uncover insights, and present findings in a compelling manner. This article explores various AI-driven tools that facilitate data analysis and visualization, providing examples of how they can be effectively implemented in academic settings.
Understanding the Role of AI in Data Analysis
AI technologies, particularly machine learning and natural language processing, enable researchers to analyze complex datasets with greater efficiency and accuracy. These tools can automate repetitive tasks, identify patterns, and generate predictive models, allowing researchers to focus on interpreting results rather than getting bogged down in data processing.
Key AI Tools for Data Analysis
- IBM Watson Studio: This platform offers a suite of tools for data scientists and researchers to build and train machine learning models. Its capabilities include data preparation, model building, and deployment, making it an invaluable resource for academic research.
- Google Cloud AutoML: Designed for users with limited machine learning expertise, Google Cloud AutoML allows researchers to train high-quality models tailored to their specific data. This tool is particularly useful for tasks such as image recognition and natural language processing.
- RapidMiner: An open-source data science platform, RapidMiner provides a user-friendly interface for data preparation, machine learning, and model evaluation. It is suitable for researchers looking to analyze data without extensive programming knowledge.
Visualizing Data with AI
Effective data visualization is crucial in academic research, as it helps convey complex findings in an understandable format. AI-driven visualization tools can automate the creation of graphs and charts, allowing researchers to present their data more effectively.
Notable AI Visualization Tools
- Tableau: While not exclusively an AI tool, Tableau incorporates AI features to enhance data visualization. Its “Ask Data” functionality allows users to pose questions in natural language, generating visualizations based on the underlying data.
- Power BI: Microsoft’s Power BI integrates AI capabilities to assist users in uncovering insights from their data. Its automated insights feature can highlight trends and anomalies, facilitating a deeper understanding of research findings.
- Qlik Sense: This analytics platform uses AI to provide intelligent visualizations and recommendations. Its associative engine allows researchers to explore data relationships dynamically, making it easier to derive insights.
Implementing AI Tools in Academic Research
To successfully implement AI tools in academic research, scholars should consider the following steps:
1. Identify Research Objectives
Clearly define the research questions and objectives to determine which AI tools will be most beneficial. This will streamline the selection process and ensure that the chosen tools align with the research goals.
2. Choose the Right Tools
Select AI tools that match the research needs, considering factors such as ease of use, scalability, and integration capabilities with existing systems.
3. Training and Support
Provide adequate training and support for researchers to familiarize themselves with the selected AI tools. This may involve workshops, online tutorials, or collaboration with data scientists.
4. Evaluate and Iterate
After implementing AI tools, continuously evaluate their effectiveness in achieving research objectives. Be open to iterating on the approach based on feedback and outcomes.
Conclusion
The adoption of AI tools for data analysis and visualization in academic research is not just a trend but a necessity in today’s data-driven environment. By utilizing the right AI-driven products, researchers can enhance their analytical capabilities, generate meaningful insights, and present their findings in a visually compelling manner. As the landscape of academic research continues to evolve, embracing these technologies will be essential for staying at the forefront of innovation.
Keyword: AI tools for academic research