AI Tools for Easy Predictive Maintenance in Energy Infrastructure
Topic: AI Coding Tools
Industry: Energy and Utilities
Explore how AI coding tools enhance predictive maintenance in energy infrastructure boosting efficiency and reducing costs for better service delivery.
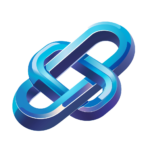
Predictive Maintenance Made Easy: AI Coding Tools for Energy Infrastructure
Understanding Predictive Maintenance in Energy Infrastructure
Predictive maintenance is a proactive approach that leverages data analytics to predict when equipment failures might occur, allowing organizations to address issues before they lead to costly downtime. In the energy and utilities sector, where infrastructure reliability is paramount, adopting predictive maintenance strategies can significantly enhance operational efficiency and reduce maintenance costs.
The Role of Artificial Intelligence in Predictive Maintenance
Artificial intelligence (AI) plays a crucial role in predictive maintenance by analyzing vast amounts of data generated by equipment and systems. AI algorithms can detect patterns and anomalies, enabling organizations to make informed decisions about maintenance schedules and resource allocation. By implementing AI-driven solutions, energy companies can transition from reactive to proactive maintenance strategies, ultimately improving service reliability and customer satisfaction.
Key AI Coding Tools for Predictive Maintenance
Several AI coding tools and platforms are available that can facilitate the implementation of predictive maintenance in energy infrastructure. Here are some noteworthy examples:
1. IBM Watson IoT
IBM Watson IoT offers a comprehensive suite of AI tools that enable organizations to connect their devices and analyze data in real-time. With its predictive analytics capabilities, Watson IoT helps energy companies monitor equipment health, predict failures, and optimize maintenance schedules, ensuring that infrastructure operates smoothly.
2. Microsoft Azure Machine Learning
Microsoft Azure Machine Learning provides a robust environment for developing and deploying predictive maintenance models. By utilizing Azure’s machine learning capabilities, energy companies can build custom algorithms that analyze historical data and predict future equipment failures, thereby minimizing unplanned outages.
3. Siemens MindSphere
Siemens MindSphere is an industrial IoT as a service solution that connects physical infrastructure to the digital world. With its advanced analytics and machine learning tools, MindSphere enables energy companies to gain insights into equipment performance, optimize maintenance operations, and enhance overall efficiency.
4. GE Predix
GE Predix is a cloud-based platform designed specifically for the industrial sector. It offers advanced analytics and machine learning capabilities that help organizations predict equipment failures and optimize maintenance workflows. By harnessing the power of Predix, energy companies can reduce operational risks and improve asset reliability.
Implementing AI-Driven Predictive Maintenance
To successfully implement AI-driven predictive maintenance, energy companies should consider the following steps:
- Data Collection: Gather historical and real-time data from equipment and sensors to create a comprehensive dataset for analysis.
- Model Development: Utilize AI coding tools to develop predictive models that can analyze the collected data and identify patterns indicative of potential failures.
- Integration: Integrate the predictive maintenance models into existing operational workflows and systems to ensure seamless functionality.
- Continuous Monitoring: Continuously monitor equipment performance and update predictive models based on new data to improve accuracy over time.
Conclusion
As the energy and utilities sector continues to evolve, the integration of AI coding tools for predictive maintenance becomes increasingly essential. By leveraging advanced analytics and machine learning, organizations can enhance their operational efficiency, reduce maintenance costs, and ultimately deliver better service to their customers. Embracing these technologies not only ensures the reliability of energy infrastructure but also positions companies for future growth in an increasingly competitive landscape.
Keyword: AI predictive maintenance tools