AI Tools for Navigating Agricultural Loans and Decision Making
Topic: AI Finance Tools
Industry: Agriculture
Discover how AI tools enhance agricultural loan decision-making by improving data analysis risk assessment and streamlining applications for farmers and businesses.
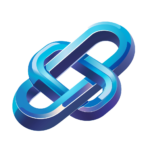
Navigating Agricultural Loans: AI Tools for Better Decision-Making
Understanding the Landscape of Agricultural Loans
Agricultural loans play a crucial role in the growth and sustainability of the farming sector. With varying requirements and conditions, navigating these loans can be a complex endeavor for farmers and agricultural businesses. In recent years, the integration of artificial intelligence (AI) in financial decision-making has emerged as a transformative approach, enabling stakeholders to make more informed choices.
The Role of AI in Agricultural Finance
AI technology can enhance the decision-making process in agricultural finance by analyzing vast amounts of data quickly and accurately. This capability allows farmers to assess their financial situations, evaluate loan options, and predict future trends with greater precision. Here are some key areas where AI can be implemented:
1. Data Analysis and Risk Assessment
AI tools can analyze historical data, market trends, and environmental factors to assess the risks associated with agricultural loans. By utilizing machine learning algorithms, these tools can identify patterns that may not be immediately apparent to human analysts. This analysis helps lenders evaluate the creditworthiness of borrowers more effectively.
2. Predictive Analytics
Predictive analytics powered by AI can forecast crop yields and market prices, providing farmers with valuable insights into their potential revenue. Tools like IBM Watson Decision Platform for Agriculture leverage AI to analyze weather patterns, soil conditions, and crop health, enabling farmers to make data-driven decisions about loan applications based on expected profitability.
3. Streamlining Loan Applications
AI-driven platforms can simplify the loan application process by automating data collection and documentation. For instance, AgriData offers a suite of tools that help farmers gather necessary financial and operational data efficiently, reducing the time spent on paperwork and increasing the speed of loan approval.
Examples of AI-Driven Tools in Agriculture
Several AI-driven products are making waves in the agricultural finance sector:
1. FarmLogs
FarmLogs is a data management platform that helps farmers track their operational performance. It utilizes AI to analyze data on crop health, weather conditions, and soil quality, allowing farmers to make informed decisions regarding their financing needs.
2. Granular
Granular provides farm management software that integrates financial data with operational metrics. Its AI capabilities help farmers understand their cost structures and profitability, which is essential for determining the right amount of financing required for growth.
3. AgFunder
AgFunder is an investment platform that uses AI to connect agricultural startups with investors. By analyzing market trends and startup performance, it helps investors make informed decisions about funding agricultural ventures, including those seeking loans.
Implementing AI Tools: A Step-by-Step Approach
For farmers and agricultural businesses looking to leverage AI tools for better decision-making regarding loans, the following steps can be beneficial:
Step 1: Assess Your Needs
Identify specific areas where AI can enhance your financial decision-making process, such as risk assessment or predictive analytics.
Step 2: Research Available Tools
Explore various AI-driven products and platforms that align with your needs. Consider factors such as ease of use, integration capabilities, and customer support.
Step 3: Implement and Train
Once you have selected the appropriate tools, implement them within your operations. Ensure that your team is trained to use these tools effectively to maximize their potential.
Step 4: Monitor and Adjust
Continuously monitor the performance of the AI tools and make adjustments as necessary. This iterative process will help you refine your decision-making capabilities over time.
Conclusion
The integration of AI tools in agricultural finance represents a significant advancement in how farmers navigate the complexities of agricultural loans. By leveraging data-driven insights, predictive analytics, and streamlined processes, stakeholders can make better-informed decisions that enhance their financial stability and growth potential. As technology continues to evolve, embracing AI in agriculture will undoubtedly pave the way for a more efficient and prosperous future.
Keyword: agricultural loans AI tools