AI Tools for Predicting Crop Yields and Profits in Farming
Topic: AI Finance Tools
Industry: Agriculture
Discover how AI forecasting tools are transforming agriculture by predicting crop yields and profits to enhance sustainability and operational efficiency for farmers
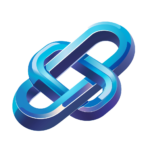
Predicting Crop Yields and Profits: The Latest AI Forecasting Tools for Farmers
Understanding the Role of AI in Agriculture
Artificial intelligence (AI) is revolutionizing various sectors, and agriculture is no exception. With the increasing pressure to enhance productivity and sustainability, farmers are turning to AI-driven tools to make informed decisions regarding crop yields and profitability. By leveraging vast amounts of data, these tools enable farmers to predict outcomes with greater accuracy, ultimately leading to improved financial performance.
Key AI Applications in Crop Yield Prediction
AI applications in agriculture primarily focus on data analysis, machine learning, and predictive modeling. These technologies help farmers assess variables such as weather patterns, soil conditions, and market trends, allowing for more precise forecasting of crop yields and associated profits.
1. Data Collection and Analysis
The first step in utilizing AI for crop yield prediction is data collection. Sensors, drones, and satellite imagery gather real-time data on soil moisture, temperature, and crop health. This information is then analyzed using AI algorithms to identify patterns and correlations.
2. Predictive Modeling
Machine learning models can be trained on historical data to predict future crop yields. By incorporating various factors such as weather forecasts and pest infestations, these models can provide insights into expected yield levels. This predictive capability allows farmers to make proactive decisions about resource allocation and crop management.
AI-Driven Tools for Farmers
Several AI-driven products have emerged in the agricultural sector, each designed to enhance yield prediction and financial forecasting. Below are some notable examples:
1. Climate Corporation
Climate Corporation offers a platform that combines weather data with agronomic insights. Their tools enable farmers to analyze field-level conditions and predict crop performance based on real-time climate data. By using their services, farmers can optimize planting schedules and manage inputs more effectively.
2. IBM Watson Decision Platform for Agriculture
IBM’s Watson Decision Platform integrates AI, weather data, and IoT devices to provide comprehensive insights into agricultural operations. This platform allows farmers to forecast yields, assess risks, and make data-driven decisions that enhance profitability.
3. Granular
Granular is a farm management software that utilizes AI to help farmers with financial planning and crop yield forecasting. By analyzing historical data and current market trends, Granular provides actionable insights that assist in maximizing profits and minimizing risks.
4. CropX
CropX combines soil sensor technology with AI to optimize irrigation and fertilization practices. By providing real-time data on soil health and moisture levels, farmers can make informed decisions that directly impact crop yields and profitability.
Implementing AI in Agricultural Practices
To successfully implement AI tools, farmers must first embrace a data-driven culture. This involves investing in technology, training staff, and fostering a mindset that prioritizes innovation. Collaboration with agricultural technology companies can also facilitate the integration of AI solutions into existing practices.
1. Training and Education
Farmers should invest in training programs to understand the capabilities and limitations of AI tools. This knowledge will empower them to leverage these technologies effectively.
2. Integration with Existing Systems
AI tools should be integrated with existing farm management systems to streamline operations. This ensures that data flows seamlessly between platforms, enhancing the accuracy of predictions.
3. Continuous Monitoring and Adjustment
AI is not a one-time solution; it requires ongoing monitoring and adjustments. Farmers should regularly review performance metrics and refine their approaches based on the insights gained from AI tools.
Conclusion
As the agriculture sector continues to evolve, the integration of AI forecasting tools is becoming increasingly essential for farmers. By predicting crop yields and profits with greater accuracy, these technologies not only enhance operational efficiency but also contribute to the overall sustainability of agricultural practices. Embracing AI-driven solutions will enable farmers to navigate the complexities of modern agriculture and secure a profitable future.
Keyword: AI crop yield prediction tools