AI Tools for Predictive Maintenance in Manufacturing Efficiency
Topic: AI Networking Tools
Industry: Manufacturing
Discover how AI networking tools enhance predictive maintenance in manufacturing by reducing downtime and optimizing maintenance schedules for better efficiency
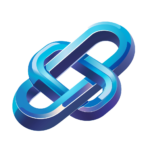
Predictive Maintenance Goes Digital: AI Networking Tools for Equipment Monitoring
Understanding Predictive Maintenance
Predictive maintenance is an advanced approach that leverages data analysis to predict when equipment failures might occur. By utilizing this strategy, manufacturers can schedule maintenance activities at optimal times, thereby reducing downtime and maintenance costs. The integration of artificial intelligence (AI) into predictive maintenance is revolutionizing the manufacturing landscape, enabling more efficient and proactive management of equipment.
The Role of AI in Predictive Maintenance
Artificial intelligence enhances predictive maintenance by analyzing vast amounts of data collected from various sources, including sensors, machinery, and operational logs. This data-driven approach allows for the identification of patterns and anomalies that may indicate potential equipment failures. AI algorithms can learn from historical data, continuously improving their predictive accuracy over time.
Key AI Networking Tools for Equipment Monitoring
Several AI-driven tools and products are available to assist manufacturers in implementing predictive maintenance effectively. Below are some noteworthy examples:
1. IBM Maximo
IBM Maximo is a comprehensive asset management solution that incorporates AI capabilities to enhance predictive maintenance. The platform utilizes machine learning algorithms to analyze equipment data, providing insights into potential failures and maintenance needs. Its predictive analytics features allow manufacturers to optimize their maintenance schedules, ultimately improving operational efficiency.
2. Siemens MindSphere
Siemens MindSphere is an industrial IoT platform that connects machines and physical infrastructure to the digital world. By harnessing AI and advanced analytics, MindSphere enables manufacturers to monitor equipment performance in real-time. The platform’s predictive maintenance applications help identify issues before they escalate, minimizing downtime and maintenance costs.
3. GE Digital’s Predix
GE Digital’s Predix platform is designed specifically for industrial applications. It offers powerful analytics capabilities that can predict equipment failures by analyzing historical and real-time data. Predix’s AI-driven insights allow manufacturers to make informed decisions regarding maintenance schedules, thereby enhancing overall productivity.
4. PTC ThingWorx
PTC ThingWorx is an IoT platform that facilitates the development of applications for smart manufacturing. Its AI capabilities enable predictive maintenance by analyzing data from connected devices. By providing manufacturers with actionable insights, ThingWorx helps optimize maintenance processes and improve asset reliability.
Implementing AI Networking Tools in Manufacturing
To successfully implement AI networking tools for predictive maintenance, manufacturers should consider the following steps:
1. Data Collection
Gathering data from various sources, including sensors and machinery, is the first step. This data serves as the foundation for AI algorithms to analyze and identify patterns.
2. Tool Selection
Choosing the right AI networking tools is crucial. Evaluate options based on specific needs, existing infrastructure, and scalability. The tools mentioned above are excellent starting points for manufacturers seeking to enhance their predictive maintenance strategies.
3. Integration
Integrating AI tools with existing systems is essential for seamless operation. Ensure that the selected tools can communicate effectively with current machinery and software.
4. Continuous Monitoring and Improvement
Once implemented, continuous monitoring of the AI tools is necessary. Regularly reviewing performance metrics and refining algorithms will help maintain accuracy and effectiveness in predictive maintenance efforts.
Conclusion
The integration of AI networking tools into predictive maintenance strategies represents a significant advancement for the manufacturing sector. By leveraging data analytics and machine learning, manufacturers can proactively manage equipment, reduce downtime, and optimize maintenance schedules. As technology continues to evolve, embracing these digital solutions will be essential for staying competitive in an increasingly complex manufacturing landscape.
Keyword: AI predictive maintenance tools