AI Tools for Risk Management in Banking Against Market Volatility
Topic: AI Finance Tools
Industry: Banking
Discover how AI tools are transforming risk management in banks enhancing fraud detection and improving resilience against market volatility
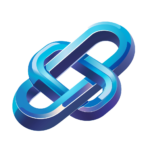
AI Tools for Risk Management: How Banks Are Staying Ahead of Market Volatility
The Role of AI in Modern Banking
In the ever-evolving landscape of finance, banks face significant challenges in managing risks associated with market volatility. The integration of artificial intelligence (AI) has emerged as a transformative solution, enabling financial institutions to enhance their risk management strategies. By leveraging AI tools, banks can proactively identify, assess, and mitigate potential risks, ensuring stability and resilience in turbulent market conditions.
Understanding Market Volatility
Market volatility refers to the fluctuations in asset prices that can be driven by various factors, including economic indicators, geopolitical events, and market sentiment. For banks, managing this volatility is crucial to maintaining customer trust and ensuring regulatory compliance. Traditional risk management methods often fall short in their ability to analyze vast amounts of data rapidly and accurately. This is where AI comes into play.
AI-Driven Risk Assessment Tools
AI-driven tools can analyze large datasets in real-time, providing banks with insights that were previously unattainable. These tools utilize machine learning algorithms to identify patterns and trends that indicate potential risks. Some notable AI tools include:
- IBM Watson: This AI platform offers advanced analytics capabilities, enabling banks to assess risk factors by analyzing historical data and predicting future trends. Its natural language processing features allow for the analysis of unstructured data, such as news articles and social media sentiment.
- Palantir Foundry: Palantir’s platform enables banks to integrate and analyze data from various sources, providing a comprehensive view of risk exposure. Its AI capabilities allow for the identification of correlations and anomalies that may indicate emerging risks.
- DataRobot: This automated machine learning platform helps banks build and deploy predictive models for risk assessment. By streamlining the model development process, DataRobot allows banks to respond more quickly to market changes.
Fraud Detection and Prevention
AI tools are also instrumental in enhancing fraud detection and prevention strategies. By analyzing transaction patterns and customer behaviors, AI can identify anomalies that may signal fraudulent activities. Examples of AI-driven fraud detection tools include:
- FICO Falcon: This AI-based solution analyzes transactions in real-time to detect fraudulent activities. By employing advanced machine learning techniques, FICO Falcon adapts to new fraud patterns, ensuring that banks remain one step ahead of potential threats.
- SAS Fraud Management: SAS utilizes AI and machine learning to provide banks with a comprehensive fraud detection system. Its ability to analyze vast amounts of data allows for the identification of suspicious activities with minimal false positives.
Stress Testing and Scenario Analysis
Stress testing is a critical component of risk management, allowing banks to evaluate their resilience under adverse market conditions. AI can enhance stress testing methodologies by providing more accurate simulations and scenario analyses. Tools that facilitate this include:
- RiskMetrics: This tool enables banks to conduct scenario analyses and stress tests by simulating various market conditions. Its AI capabilities enhance the accuracy of predictions, helping banks to prepare for potential downturns.
- Moody’s Analytics: Moody’s offers advanced risk modeling solutions that incorporate AI to improve the precision of stress testing. By analyzing historical data and market trends, banks can better understand their risk exposure and make informed decisions.
Implementing AI in Risk Management
For banks looking to implement AI tools in their risk management strategies, several steps can be taken:
1. Data Integration
Successful AI implementation begins with the integration of diverse data sources. Banks must ensure that they have access to high-quality, structured, and unstructured data to feed into AI models.
2. Selecting the Right Tools
Choosing the appropriate AI tools is crucial. Banks should evaluate their specific needs and objectives, considering factors such as scalability, ease of use, and integration capabilities.
3. Continuous Monitoring and Adaptation
The financial landscape is dynamic, and AI models must be continuously monitored and updated to reflect changing market conditions. Regular assessments will ensure that the tools remain effective in identifying and managing risks.
Conclusion
As market volatility continues to pose challenges for banks, the adoption of AI tools for risk management is becoming increasingly essential. By leveraging advanced analytics, machine learning, and predictive modeling, financial institutions can enhance their risk assessment capabilities, improve fraud detection, and conduct more effective stress testing. Embracing these AI-driven solutions will not only help banks navigate turbulent markets but also position them for sustained success in the future.
Keyword: AI tools for risk management