AI Tools Transforming Demand Forecasting in Retail Analytics
Topic: AI News Tools
Industry: Retail and E-commerce
Discover how AI tools like IBM Watson and Microsoft Azure are transforming demand forecasting in retail with predictive analytics for better accuracy and efficiency
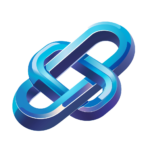
Predictive Analytics in Retail: AI Tools Shaping the Future of Demand Forecasting
Understanding Predictive Analytics
Predictive analytics refers to the use of statistical algorithms and machine learning techniques to identify the likelihood of future outcomes based on historical data. In the retail sector, this approach is increasingly essential for demand forecasting, enabling businesses to make informed decisions about inventory management, pricing strategies, and marketing efforts.
The Role of AI in Demand Forecasting
Artificial intelligence (AI) enhances predictive analytics by processing vast amounts of data at unprecedented speeds. Retailers can leverage AI to analyze customer behavior, seasonal trends, and external factors, allowing for more accurate demand predictions. The integration of AI tools not only improves forecasting accuracy but also optimizes supply chain operations and enhances customer satisfaction.
Key AI Tools and Technologies
Several AI-driven tools are revolutionizing demand forecasting in retail. Below are some notable examples:
1. IBM Watson Analytics
IBM Watson Analytics offers advanced data analysis capabilities that allow retailers to visualize trends and patterns in their sales data. By utilizing natural language processing, users can query their data using everyday language, making it accessible for non-technical staff. This tool helps retailers forecast demand by analyzing customer preferences and purchasing behaviors.
2. Microsoft Azure Machine Learning
Microsoft Azure Machine Learning provides a robust platform for building predictive models. Retailers can use this tool to create custom algorithms that analyze historical sales data, weather patterns, and economic indicators. Azure’s scalability allows businesses to adjust their forecasting models as new data becomes available, ensuring that predictions remain accurate and relevant.
3. Google Cloud AI
Google Cloud AI offers a suite of machine learning tools that can be utilized for demand forecasting. Retailers can harness Google’s powerful data processing capabilities to analyze customer interactions across multiple channels. With features like AutoML, businesses can build and deploy custom machine learning models without requiring extensive coding knowledge, making it easier to implement predictive analytics.
Implementing AI in Demand Forecasting
To successfully implement AI-driven predictive analytics, retailers should consider the following steps:
1. Data Collection and Integration
Gathering relevant data from various sources, such as POS systems, e-commerce platforms, and customer relationship management (CRM) systems, is crucial. Integration of these data sources into a centralized platform will facilitate comprehensive analysis.
2. Model Development
Retailers should collaborate with data scientists to develop predictive models tailored to their specific needs. This involves selecting appropriate algorithms and training the models using historical data to improve accuracy.
3. Continuous Monitoring and Adjustment
Once implemented, it is essential to continuously monitor the performance of the predictive models. Retailers should be prepared to adjust their models based on new data and changing market conditions to maintain accuracy in demand forecasting.
Benefits of AI-Driven Demand Forecasting
The adoption of AI tools for demand forecasting offers numerous benefits for retailers:
- Enhanced Accuracy: AI algorithms can analyze complex datasets to produce more accurate forecasts than traditional methods.
- Cost Efficiency: Improved demand predictions lead to optimized inventory levels, reducing holding costs and minimizing stockouts or overstock situations.
- Informed Decision-Making: Retailers can make strategic decisions regarding product launches, promotions, and pricing based on reliable forecasts.
Conclusion
As the retail landscape continues to evolve, the integration of AI-driven predictive analytics is becoming increasingly vital for effective demand forecasting. By leveraging advanced tools such as IBM Watson Analytics, Microsoft Azure Machine Learning, and Google Cloud AI, retailers can enhance their forecasting accuracy, optimize operations, and ultimately improve customer satisfaction. Embracing these technologies will not only shape the future of demand forecasting but also secure a competitive edge in the dynamic retail market.
Keyword: AI tools for demand forecasting