AI Transforming Fraud Detection in Banking by 2025
Topic: AI Other Tools
Industry: Finance and Banking
Discover how AI is transforming fraud detection in banking with enhanced data analysis predictive analytics and automated decision-making for 2025
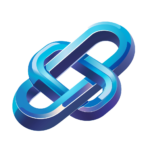
5 Ways AI is Revolutionizing Fraud Detection in Banking for 2025
1. Enhanced Data Analysis
Artificial Intelligence (AI) is transforming the way banks analyze vast amounts of data. Traditional methods of fraud detection often rely on historical data and predefined rules, which can be easily circumvented by sophisticated fraudsters. In contrast, AI-driven tools utilize machine learning algorithms to analyze real-time data from multiple sources, identifying patterns and anomalies that may indicate fraudulent activity.
Implementation Example: SAS Fraud Management
SAS Fraud Management employs advanced analytics and machine learning to detect and prevent fraud in real-time. By continuously learning from new data, it adapts to emerging fraud trends, allowing banks to respond proactively rather than reactively.
2. Predictive Analytics for Proactive Measures
AI enables banks to employ predictive analytics, which can forecast potential fraud before it occurs. By analyzing customer behavior and transaction history, AI systems can identify high-risk transactions and flag them for further investigation.
Implementation Example: FICO Falcon Fraud Manager
The FICO Falcon Fraud Manager uses predictive analytics to assess risk levels for each transaction. By leveraging historical data and machine learning, it can predict the likelihood of fraud, allowing banks to take preventive measures before a transaction is completed.
3. Natural Language Processing for Customer Interactions
Natural Language Processing (NLP) is another AI capability that enhances fraud detection. By analyzing customer communications, such as emails and chat messages, banks can identify potential fraud attempts based on language patterns and sentiment analysis.
Implementation Example: IBM Watson
IBM Watson’s NLP capabilities can be integrated into customer service platforms to monitor communications for signs of fraud. By flagging suspicious interactions, banks can investigate further and mitigate risks before they escalate.
4. Automated Decision-Making
AI facilitates automated decision-making processes, allowing banks to respond to potential fraud more swiftly. By utilizing AI algorithms, institutions can automate the approval or denial of transactions based on risk assessments, significantly reducing the time it takes to address fraud.
Implementation Example: Zest AI
Zest AI offers a platform that automates credit decisions using machine learning. By integrating fraud detection into the decision-making process, banks can enhance their operational efficiency while minimizing the risk of fraudulent transactions.
5. Continuous Learning and Adaptation
One of the most significant advantages of AI in fraud detection is its ability to continuously learn and adapt to new fraud techniques. As fraudsters evolve their tactics, AI systems can be updated with new data, ensuring that banks remain one step ahead.
Implementation Example: Darktrace
Darktrace employs self-learning AI to detect and respond to cyber threats in real-time. Its ability to adapt to new threats makes it an invaluable tool for banks looking to enhance their fraud detection capabilities.
Conclusion
As we move towards 2025, the integration of AI in fraud detection is not merely an option but a necessity for banks aiming to safeguard their operations and customers. By leveraging advanced data analysis, predictive analytics, natural language processing, automated decision-making, and continuous learning, financial institutions can significantly enhance their fraud detection capabilities. Investing in these AI-driven tools will not only help mitigate risks but also foster greater trust and security among customers.
Keyword: AI fraud detection in banking