AI Transforming Predictive Maintenance in Manufacturing Strategies
Topic: AI Other Tools
Industry: Manufacturing
Discover how AI is transforming predictive maintenance in manufacturing through enhanced data analysis real-time monitoring and improved resource allocation.
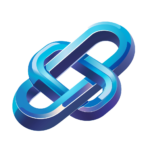
5 Ways AI is Revolutionizing Predictive Maintenance in Manufacturing
1. Enhanced Data Analysis
Artificial intelligence (AI) has the capability to process vast amounts of data far beyond human capacity. In manufacturing, AI-driven tools analyze historical performance data from machinery to identify patterns and predict potential failures before they occur. Tools like Predictive Analytics for Manufacturing by IBM utilize machine learning algorithms to sift through operational data, enabling manufacturers to make informed decisions based on predictive insights.
2. Real-Time Monitoring
Implementing AI in predictive maintenance allows manufacturers to monitor equipment in real-time. Systems equipped with sensors can collect data on various parameters such as temperature, vibration, and pressure. AI platforms, such as Uptake, leverage this data to provide real-time insights and alerts, allowing maintenance teams to address issues proactively, thereby minimizing downtime and extending equipment lifespan.
3. Improved Resource Allocation
AI can optimize resource allocation by predicting when maintenance will be required, allowing companies to schedule repairs during non-peak hours. This not only reduces production interruptions but also helps in managing labor costs more effectively. Tools like Fiix offer AI-driven maintenance scheduling that aligns workforce availability with predicted equipment needs, ensuring that resources are deployed efficiently.
4. Integration with IoT Devices
The integration of AI with Internet of Things (IoT) devices is transforming predictive maintenance strategies. IoT devices collect real-time data from machinery, which AI algorithms can analyze to forecast failures. For instance, GE Digital’s Predix platform combines IoT data with AI analytics to create a comprehensive picture of equipment health, enabling manufacturers to implement maintenance strategies that are both timely and effective.
5. Continuous Learning and Improvement
One of the most significant advantages of AI in predictive maintenance is its ability to learn and adapt over time. As more data is collected, AI systems refine their algorithms to improve accuracy in predicting equipment failures. Platforms like Siemens MindSphere utilize machine learning to continuously enhance their predictive capabilities, ensuring that manufacturers can stay ahead of potential issues and maintain operational efficiency.
Conclusion
The integration of AI into predictive maintenance is not just a trend; it is a necessity for manufacturers aiming to enhance efficiency and reduce operational costs. By leveraging advanced tools and technologies, companies can transform their maintenance strategies, ensuring they remain competitive in an increasingly demanding market. As AI continues to evolve, its impact on predictive maintenance will only grow, paving the way for smarter, more efficient manufacturing processes.
Keyword: AI predictive maintenance manufacturing