AI Transforming Telecom Infrastructure with Predictive Maintenance
Topic: AI Analytics Tools
Industry: Telecommunications
Discover how AI-driven predictive maintenance is transforming telecom infrastructure by reducing downtime and costs while enhancing service reliability and customer satisfaction.
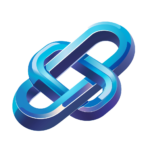
Predictive Maintenance Revolution: How AI is Transforming Telecom Infrastructure
The Emergence of Predictive Maintenance in Telecommunications
As the telecommunications industry continues to expand and evolve, the need for efficient maintenance practices has never been more critical. Predictive maintenance, powered by artificial intelligence (AI), is revolutionizing how telecom operators manage their infrastructure. By leveraging AI analytics tools, companies can anticipate equipment failures, optimize operations, and ultimately enhance service delivery.
Understanding Predictive Maintenance
Predictive maintenance refers to the use of data analysis and AI algorithms to predict when equipment failures might occur. This proactive approach allows telecommunications companies to perform maintenance activities just in time, reducing downtime and operational costs. By analyzing historical data and real-time metrics, AI can identify patterns and anomalies that indicate potential issues.
Benefits of AI-Driven Predictive Maintenance
- Reduced Downtime: By predicting failures before they occur, telecom operators can minimize service interruptions.
- Cost Efficiency: Targeted maintenance reduces unnecessary repairs and extends the lifespan of equipment.
- Enhanced Customer Satisfaction: Improved reliability leads to better service quality and customer retention.
Implementing AI in Predictive Maintenance
To effectively implement AI-driven predictive maintenance, telecommunications companies must integrate several key components:
Data Collection and Integration
Gathering data from various sources, including network performance metrics, equipment logs, and environmental conditions, is the first step. Tools such as Splunk and IBM Watson IoT can facilitate this data collection and integration process, creating a comprehensive view of the infrastructure.
AI Analytics Tools
Once data is collected, AI analytics tools come into play. These tools utilize machine learning algorithms to analyze large datasets, identify trends, and predict future failures. Examples of effective AI-driven products include:
- GE Digital’s Predix: This platform offers advanced analytics capabilities specifically designed for industrial IoT, enabling telecom operators to monitor equipment health in real-time.
- Siemens MindSphere: An open cloud-based IoT operating system that connects physical products to the digital world, providing insights that help in predictive maintenance.
- Microsoft Azure IoT: This cloud service provides tools for building and deploying predictive maintenance solutions, allowing telecom companies to harness the power of AI without extensive infrastructure investment.
Continuous Monitoring and Feedback Loop
Implementing a continuous monitoring system is essential. Tools like Tableau and Power BI can visualize data trends and performance metrics, enabling operators to make informed decisions based on real-time insights. Establishing a feedback loop ensures that the AI models are continuously refined based on new data, improving their accuracy over time.
Case Studies: AI in Action
Several telecommunications companies have already begun harnessing the power of AI for predictive maintenance:
AT&T
AT&T has implemented AI-driven predictive maintenance systems to monitor its network infrastructure, significantly reducing outages and improving service reliability. By analyzing data from their network equipment, they can predict potential failures and address them proactively.
Vodafone
Vodafone has utilized AI analytics tools to enhance its maintenance strategies, resulting in a 30% reduction in maintenance costs. Their approach combines machine learning with real-time data analysis to optimize their network performance.
Conclusion
The adoption of AI-driven predictive maintenance is not merely a trend but a necessity for telecommunications companies aiming to stay competitive in a rapidly evolving market. By leveraging advanced analytics tools and implementing robust data strategies, telecom operators can achieve substantial improvements in operational efficiency, cost savings, and customer satisfaction. As the technology continues to advance, the potential for AI in telecommunications will only grow, paving the way for a more reliable and efficient industry.
Keyword: AI predictive maintenance telecom