AI Triage Solutions for Efficient Imaging Center Operations
Topic: AI Health Tools
Industry: Diagnostic imaging centers
Discover how AI-powered triage enhances efficiency in imaging centers by prioritizing critical cases and improving patient care in a demanding healthcare landscape
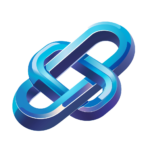
AI-Powered Triage: Prioritizing Critical Cases in Busy Imaging Centers
The Increasing Demand for Efficient Imaging Services
As healthcare systems worldwide face mounting pressure to deliver timely and accurate diagnoses, imaging centers are experiencing a significant surge in demand. This escalation not only strains resources but also raises the stakes for patient outcomes, particularly for critical cases requiring immediate attention. In this context, the integration of artificial intelligence (AI) tools in diagnostic imaging centers emerges as a transformative solution to enhance efficiency and prioritize urgent cases effectively.
Understanding AI in Diagnostic Imaging
Artificial intelligence in diagnostic imaging refers to the use of algorithms and machine learning techniques to analyze medical images, assist radiologists, and streamline workflows. By harnessing the power of AI, imaging centers can reduce turnaround times for critical cases, improve diagnostic accuracy, and ultimately enhance patient care.
Key Benefits of AI-Powered Triage
- Improved Efficiency: AI algorithms can process and analyze vast amounts of imaging data much faster than human radiologists, allowing for quicker identification of critical cases.
- Enhanced Accuracy: AI tools can assist in reducing human error by providing second opinions and flagging abnormalities that may be overlooked in a busy setting.
- Resource Optimization: By prioritizing critical cases, AI helps imaging centers allocate their resources more effectively, ensuring that urgent cases receive the attention they need without overwhelming staff.
Implementing AI Tools in Imaging Centers
To successfully integrate AI into imaging workflows, diagnostic centers must consider several key components:
1. Selecting the Right AI Tools
Choosing the appropriate AI-driven products is crucial for effective implementation. Some notable examples include:
- RadNet: This AI platform utilizes deep learning algorithms to analyze radiological images, helping to prioritize cases based on urgency and potential severity.
- Aidoc: Aidoc offers real-time analysis of CT scans, automatically flagging critical findings such as intracranial hemorrhages or pulmonary embolisms, allowing radiologists to focus on urgent cases first.
- Qure.ai: This tool leverages AI to interpret chest X-rays and CT scans, providing automated reports that highlight critical abnormalities and streamline triage processes.
2. Training Staff and Ensuring Collaboration
Implementing AI tools requires training staff to understand and effectively utilize these technologies. Radiologists and technicians should be well-versed in the capabilities and limitations of AI systems to foster a collaborative environment where human expertise and AI insights work in tandem.
3. Continuous Monitoring and Evaluation
Once AI tools are integrated into the workflow, continuous monitoring is essential to evaluate their performance and impact on patient outcomes. Imaging centers should establish metrics to assess the accuracy of AI predictions and the efficiency of triage processes, ensuring that the technology evolves to meet changing demands.
Case Studies: Successful Implementation of AI in Imaging
Several imaging centers have successfully integrated AI-powered triage systems, yielding significant improvements in patient care:
Example 1: A Leading Hospital Network
A prominent hospital network implemented Aidoc’s AI solution across its radiology departments. Within six months, the network reported a 30% reduction in turnaround time for critical cases, allowing radiologists to prioritize life-threatening conditions more effectively.
Example 2: Community Imaging Center
A community imaging center adopted Qure.ai’s chest X-ray analysis tool, which led to a 25% increase in the identification of critical cases. The center was able to streamline its workflow, ensuring that patients with urgent needs received timely interventions.
Conclusion
As the demand for imaging services continues to rise, AI-powered triage systems present a viable solution for imaging centers striving to enhance efficiency and prioritize critical cases. By implementing the right AI tools, training staff, and continuously monitoring outcomes, diagnostic imaging centers can make significant strides in improving patient care while managing the complexities of a busy healthcare environment.
Keyword: AI triage in imaging centers