AI Weather Tools Enhancing Energy Distribution and Storage
Topic: AI Weather Tools
Industry: Energy and Utilities
Discover how AI weather tools optimize energy distribution and storage by enhancing forecasting accuracy and enabling real-time decision-making in the energy sector
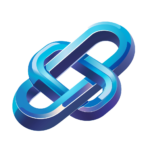
Beyond Forecasting: AI Weather Tools for Optimizing Energy Distribution and Storage
The Intersection of AI and Weather Data in Energy Management
As the energy sector continues to evolve, the integration of artificial intelligence (AI) with weather data presents unprecedented opportunities for optimizing energy distribution and storage. Traditional forecasting methods, while useful, often fall short in providing the granularity and real-time responsiveness required for modern energy management. AI weather tools are stepping in to bridge this gap, offering utilities and energy providers enhanced capabilities to make data-driven decisions.
Understanding AI Weather Tools
AI weather tools leverage machine learning algorithms to analyze vast amounts of meteorological data, including temperature, humidity, wind patterns, and solar radiation. By processing this information, these tools can predict energy demand and generation with remarkable accuracy. This predictive capability is crucial for utilities aiming to balance supply and demand efficiently.
Key Features of AI Weather Tools
- Real-Time Data Analysis: AI tools can process real-time weather data, allowing for immediate adjustments in energy distribution strategies.
- Predictive Analytics: By utilizing historical weather patterns and current data, AI can forecast energy production from renewable sources, such as solar and wind.
- Scenario Modeling: These tools can simulate various weather scenarios, helping utilities prepare for potential disruptions and optimize their response strategies.
Implementing AI Weather Tools in Energy Distribution
To effectively implement AI weather tools, energy providers must consider several key aspects:
1. Data Integration
Successful deployment of AI weather tools requires seamless integration with existing data management systems. This includes historical energy usage data, grid performance metrics, and real-time weather information. By creating a comprehensive data ecosystem, utilities can enhance the predictive capabilities of AI tools.
2. Collaboration with Technology Providers
Partnering with technology firms specializing in AI and weather analytics can accelerate the implementation process. Companies like IBM and Siemens offer AI-driven solutions tailored for the energy sector. For instance, IBM’s Weather Company provides advanced analytics that can be integrated with energy management systems to optimize grid operations.
3. Continuous Learning and Adaptation
AI systems thrive on continuous learning. By regularly updating algorithms with new data, utilities can improve the accuracy of their forecasts and adapt to changing weather patterns. This iterative process ensures that energy distribution remains efficient and responsive to real-time conditions.
Examples of AI-Driven Products
Several AI-driven products are currently making waves in the energy sector, enhancing the capabilities of utilities in managing weather-related challenges:
1. Enel’s AI Weather Forecasting Tool
Enel, a multinational energy company, has developed an AI tool that utilizes machine learning to forecast solar and wind energy production. By analyzing weather forecasts and historical data, the tool provides accurate predictions that allow for better energy distribution and storage management.
2. Google’s DeepMind Energy Optimization
Google’s DeepMind has collaborated with energy providers to optimize energy usage in data centers. By applying AI to weather data, the system can predict cooling needs based on upcoming weather conditions, significantly reducing energy consumption and operational costs.
3. Uplight’s Demand Response Solutions
Uplight offers AI-driven demand response solutions that adjust energy consumption based on real-time weather forecasts. This not only helps in balancing the grid but also empowers consumers to manage their energy usage more effectively during peak demand periods.
Conclusion
As the energy sector faces increasing pressure to optimize operations and reduce carbon footprints, AI weather tools emerge as a vital asset. By moving beyond traditional forecasting methods, utilities can harness the power of AI to enhance energy distribution and storage strategies. The integration of these advanced tools not only improves operational efficiency but also supports the transition towards a more sustainable energy future.
Keyword: AI weather tools for energy management