Anonymizing Driver Data with AI for Automotive Privacy
Topic: AI Privacy Tools
Industry: Automotive
Discover AI techniques for anonymizing driver data in the automotive industry ensuring privacy compliance while gaining valuable analytics insights.
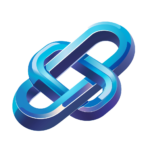
Anonymizing Driver Data: AI Techniques for Privacy-First Automotive Analytics
Understanding the Importance of Data Privacy in the Automotive Industry
As the automotive industry increasingly embraces connectivity and automation, the collection of driver data has become a fundamental aspect of enhancing user experience and improving vehicle performance. However, this surge in data acquisition raises significant privacy concerns. Protecting sensitive driver information is paramount, not only to comply with regulations such as GDPR and CCPA but also to build consumer trust.
AI-Driven Anonymization Techniques
Artificial Intelligence (AI) offers innovative solutions for anonymizing driver data, ensuring that privacy is prioritized while still enabling valuable analytics. Here are several AI techniques that can be implemented:
1. Data Masking
Data masking involves altering identifiable information within a dataset to prevent the identification of individuals. AI algorithms can automate the process of data masking by recognizing patterns and replacing sensitive data with fictional or scrambled values. This ensures that analytics can still be performed without exposing personal information.
2. Differential Privacy
Differential privacy is a mathematical framework that enables organizations to extract insights from data while providing strong privacy guarantees. By adding controlled noise to the data, AI systems can ensure that the output does not reveal any information about individual drivers. This technique can be particularly useful in aggregating driving behavior data for trend analysis without compromising individual privacy.
3. Federated Learning
Federated learning is an AI technique that allows models to be trained across decentralized devices without the need to share raw data. Instead of sending personal data to a central server, the AI model is trained locally on the driver’s device, and only the model updates are shared. This approach significantly enhances data privacy, as sensitive information never leaves the driver’s device.
Examples of AI-Driven Privacy Tools
Several tools and platforms are leading the way in implementing AI-driven privacy solutions within the automotive sector:
1. IBM Watson for Automotive
IBM Watson provides a suite of AI tools that can assist automotive companies in managing and anonymizing driver data. With capabilities in natural language processing and machine learning, IBM Watson can analyze vast amounts of data while ensuring compliance with privacy regulations through advanced anonymization techniques.
2. Google Cloud’s Differential Privacy
Google Cloud offers a differential privacy toolkit that enables organizations to analyze data while preserving individual privacy. This tool can be integrated into automotive analytics platforms to help manufacturers gain insights from driver data without compromising personal information.
3. Tonic.ai
Tonic.ai specializes in data synthesis and anonymization, providing a platform that generates realistic synthetic data based on existing datasets. This allows automotive companies to conduct testing and analysis without exposing real driver data, thereby maintaining privacy and compliance.
Conclusion
As the automotive industry continues to evolve, the importance of protecting driver data cannot be overstated. By leveraging AI techniques for anonymization, companies can ensure that they uphold privacy standards while still gaining valuable insights from data analytics. Implementing tools such as IBM Watson, Google Cloud’s Differential Privacy, and Tonic.ai can help automotive organizations navigate the complex landscape of data privacy, fostering a culture of trust and transparency with their customers.
Keyword: AI techniques for driver data privacy