Automating Supplier Performance Evaluation with Machine Learning
Topic: AI Relationship Tools
Industry: Logistics and Supply Chain
Automate supplier performance evaluation with machine learning to gain data-driven insights enhance accuracy and reduce bias for better supply chain management
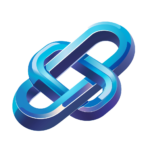
Automating Supplier Performance Evaluation with Machine Learning
Understanding the Importance of Supplier Performance Evaluation
In today’s fast-paced logistics and supply chain environment, the ability to evaluate supplier performance effectively is crucial for maintaining competitive advantage. As organizations strive to optimize their supply chains, the traditional methods of supplier evaluation often fall short. Manual assessments can be time-consuming, prone to bias, and may not provide the depth of analysis needed for informed decision-making.
The Role of Machine Learning in Supplier Evaluation
Machine learning (ML) offers a transformative approach to automating supplier performance evaluation. By leveraging vast amounts of data, ML algorithms can identify patterns and insights that would be difficult to discern through manual processes. This not only enhances the accuracy of evaluations but also enables organizations to respond proactively to supplier-related challenges.
Key Benefits of Machine Learning in Supplier Performance Evaluation
- Data-Driven Insights: ML algorithms analyze historical performance data, allowing businesses to gain insights into supplier reliability, quality, and delivery times.
- Real-Time Monitoring: Continuous data collection and analysis enable organizations to monitor supplier performance in real-time, facilitating timely interventions when issues arise.
- Reduced Bias: By relying on data rather than subjective assessments, ML minimizes bias in supplier evaluations, leading to more objective decision-making.
Implementing AI in Supplier Evaluation
To effectively implement AI-driven solutions for supplier performance evaluation, organizations must consider several key steps:
1. Data Collection
Gathering relevant data from various sources is the foundation of any successful machine learning initiative. This includes historical performance data, contract compliance metrics, and customer feedback.
2. Choosing the Right Tools
There are several AI-driven products and tools available that can facilitate supplier performance evaluation:
- IBM Watson Supply Chain: This tool uses AI to provide insights into supplier performance, risk assessment, and overall supply chain optimization.
- Oracle Procurement Cloud: Leveraging machine learning, this platform helps organizations automate supplier evaluations and track performance metrics effectively.
- Coupa: Coupa’s AI capabilities enable organizations to analyze supplier data, identify trends, and automate performance reviews.
3. Training the Model
Once the data is collected and the tools are selected, organizations must train their machine learning models. This involves feeding the model historical data to help it recognize patterns and make predictions about future supplier performance.
4. Continuous Improvement
Machine learning models require ongoing refinement. As new data becomes available, organizations should continuously update their models to improve accuracy and relevance.
Case Study: Successful Implementation of Machine Learning in Supplier Evaluation
A leading automotive manufacturer recently implemented a machine learning solution to enhance its supplier performance evaluation process. By utilizing a combination of IBM Watson and custom-built algorithms, the company was able to automate the evaluation of over 200 suppliers. The results were significant:
- Improved supplier reliability by 30% within the first year.
- Reduced time spent on manual evaluations by 50%.
- Enhanced ability to predict supplier-related risks, allowing for proactive management.
Conclusion
As logistics and supply chain operations become increasingly complex, the automation of supplier performance evaluation through machine learning presents a significant opportunity for organizations to enhance efficiency and effectiveness. By implementing AI-driven tools, businesses can gain valuable insights, reduce bias, and ultimately foster stronger supplier relationships. The future of supplier evaluation lies in the intelligent application of technology, and organizations that embrace this shift will be well-positioned for success.
Keyword: automating supplier performance evaluation