Battle of Algorithms AI Recommendation Systems in Streaming
Topic: AI Music Tools
Industry: Streaming Services
Explore the battle of AI recommendation systems among major streaming platforms and discover how they shape music discovery and user experience in the digital age
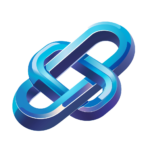
The Battle of Algorithms: Comparing AI Recommendation Systems Across Major Streaming Platforms
Understanding AI Recommendation Systems
In the rapidly evolving landscape of digital music consumption, streaming platforms have increasingly turned to artificial intelligence (AI) to enhance user experience. AI recommendation systems play a crucial role in shaping how users discover music, tailoring suggestions based on individual preferences and listening habits. As competition intensifies among major players, understanding the nuances of these algorithms becomes essential for both consumers and industry stakeholders.
Key Players in the Streaming Arena
Several streaming platforms have made significant investments in AI-driven recommendation systems. Notable players include:
- Spotify: Known for its sophisticated algorithms, Spotify utilizes a combination of collaborative filtering and natural language processing to curate personalized playlists such as “Discover Weekly” and “Release Radar.”
- Apple Music: Apple Music leverages machine learning to analyze user behavior and preferences, integrating human curators to enhance its recommendations.
- YouTube Music: By using deep learning techniques, YouTube Music analyzes user interactions and video metadata to suggest songs and playlists that resonate with listeners.
How AI is Implemented in Music Recommendations
The implementation of AI in recommendation systems generally involves several key components:
Data Collection
Streaming services collect vast amounts of data, including user listening history, search queries, and even social media interactions. This data serves as the foundation for training AI algorithms.
Machine Learning Algorithms
Machine learning models, such as collaborative filtering and content-based filtering, are employed to analyze user data. Collaborative filtering identifies patterns among users with similar tastes, while content-based filtering focuses on the attributes of the music itself.
Continuous Learning
AI systems are designed to continuously learn and adapt. As users interact with the platform, the algorithms refine their recommendations, ensuring that they remain relevant and engaging over time.
Examples of AI-Driven Tools and Products
Several AI-driven tools enhance the recommendation capabilities of streaming platforms, including:
1. Echo Nest (Acquired by Spotify)
Echo Nest provides advanced music intelligence solutions that analyze song attributes, user preferences, and social media trends. Its technology powers Spotify’s recommendation engine, allowing for highly personalized listening experiences.
2. Amper Music
Amper Music is an AI-driven music composition tool that enables users to create custom music tracks. While it primarily focuses on content creation, it also showcases how AI can be utilized to enhance user engagement within streaming platforms.
3. AIVA (Artificial Intelligence Virtual Artist)
AIVA is an AI composer that generates original music. Its integration in streaming services can provide unique soundtracks for playlists, enhancing the overall listening experience.
Challenges and Considerations
Despite the advancements in AI recommendation systems, several challenges persist:
Data Privacy
As streaming services gather extensive user data, ensuring privacy and compliance with regulations such as GDPR is paramount. Balancing personalization with user consent remains a critical issue.
Algorithmic Bias
AI systems can inadvertently perpetuate biases present in the training data. Streaming platforms must remain vigilant to ensure that their recommendations do not favor certain genres or artists disproportionately.
The Future of AI in Music Streaming
As AI technology continues to evolve, the potential for innovation in music recommendation systems is vast. Future developments may include:
- Enhanced personalization through emotion recognition and sentiment analysis.
- Integration of augmented reality (AR) and virtual reality (VR) experiences that adapt based on user preferences.
- Collaborative playlists created by AI that reflect global music trends while catering to local tastes.
Conclusion
The battle of algorithms among major streaming platforms underscores the pivotal role of AI in shaping the music listening experience. As these technologies advance, they will not only redefine how users discover music but also influence the broader music industry landscape. Stakeholders must remain informed and adaptable to harness the full potential of AI-driven recommendation systems.
Keyword: AI music recommendation systems