Early Pest and Disease Detection in Crops Using Machine Learning
Topic: AI Analytics Tools
Industry: Agriculture
Discover how machine learning enhances early pest and disease detection in crops improving productivity and sustainability in agriculture
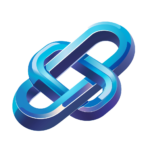
Leveraging Machine Learning for Early Pest and Disease Detection in Crops
Introduction to AI in Agriculture
The agricultural sector is experiencing a transformative shift due to advancements in artificial intelligence (AI) and machine learning (ML). These technologies are not only enhancing productivity but also ensuring sustainable farming practices. One of the most significant applications of AI in agriculture is the early detection of pests and diseases, which can lead to timely interventions and reduced crop losses.
The Importance of Early Detection
Early detection of pests and diseases is crucial for maintaining crop health and maximizing yield. Traditional methods of monitoring crops can be labor-intensive and often rely on human expertise, which may not always be available. By integrating AI analytics tools, farmers can automate the detection process, enabling them to act swiftly and effectively.
How Machine Learning Works in Pest and Disease Detection
Machine learning algorithms analyze data collected from various sources, such as satellite imagery, drone footage, and on-ground sensors. These algorithms can identify patterns and anomalies that indicate the presence of pests or diseases. Over time, as more data is fed into the system, the algorithms become increasingly accurate in their predictions.
Implementation of AI in Crop Monitoring
Implementing AI-driven solutions for pest and disease detection involves several steps:
- Data Collection: Utilize sensors, drones, and satellite imagery to gather data on crop health, soil conditions, and environmental factors.
- Data Processing: Use AI analytics tools to process and analyze the collected data, identifying patterns that may indicate pest infestations or disease outbreaks.
- Real-Time Monitoring: Implement systems that provide real-time alerts to farmers, enabling them to respond promptly to potential threats.
Examples of AI-Driven Tools for Pest and Disease Detection
Several AI-driven products and tools are currently available that exemplify the effective use of machine learning in agriculture:
1. Plantix
Plantix is a mobile application that employs image recognition technology to diagnose plant diseases and pest infestations. Farmers can take photos of their crops, and the app analyzes the images to provide immediate feedback and suggested treatments. This tool empowers farmers with quick access to critical information, enhancing their decision-making capabilities.
2. Taranis
Taranis is a precision agriculture platform that utilizes high-resolution aerial imagery and AI analytics to monitor crop health. The platform can detect pest infestations and diseases early by analyzing the imagery for signs of stress or damage. Taranis also offers actionable insights, allowing farmers to apply targeted interventions.
3. AgroStar
AgroStar combines AI with agronomic expertise to provide farmers with tailored recommendations for pest and disease management. Through its mobile app, farmers receive alerts about potential threats based on real-time data analysis, enabling them to take proactive measures to protect their crops.
Challenges and Considerations
While the benefits of leveraging machine learning for pest and disease detection are substantial, there are challenges to consider:
- Data Quality: The accuracy of machine learning models depends heavily on the quality of the data collected. Ensuring high-quality, relevant data is essential for effective analysis.
- Integration with Existing Systems: Farmers may face difficulties integrating new AI tools with their existing agricultural practices and technologies.
- Cost of Implementation: The initial investment in AI technologies can be significant, and farmers must weigh the potential return on investment.
Conclusion
Leveraging machine learning for early pest and disease detection in crops presents a significant opportunity for farmers to enhance productivity and sustainability. By implementing AI analytics tools, the agricultural sector can move towards more efficient and effective crop management practices. As technology continues to evolve, the potential for AI in agriculture will only grow, paving the way for a more resilient food system.
Keyword: early pest detection in crops