Enhance Supply Chain Resilience with AI Analytics and Forecasting
Topic: AI News Tools
Industry: Manufacturing
Enhance supply chain resilience with AI-driven analytics and forecasting Discover how AI tools improve decision-making and operational efficiency for manufacturers
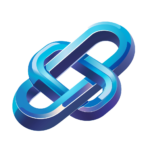
Enhancing Supply Chain Resilience with AI-Driven Analytics and Forecasting
The Importance of Supply Chain Resilience
In today’s rapidly evolving market landscape, supply chain resilience has become a critical focus for manufacturers. Disruptions from global events, natural disasters, and market fluctuations can significantly impact operational efficiency and profitability. To mitigate these risks, organizations are increasingly turning to artificial intelligence (AI) for enhanced analytics and forecasting capabilities.
Leveraging AI for Enhanced Analytics
AI-driven analytics enable manufacturers to process vast amounts of data quickly and accurately, uncovering insights that can inform decision-making. By integrating AI tools into their supply chain operations, manufacturers can achieve greater visibility and responsiveness.
Key AI Tools for Supply Chain Analytics
- IBM Watson Supply Chain: This platform utilizes AI to analyze supply chain data, offering predictive insights that help businesses anticipate disruptions and optimize inventory levels.
- Microsoft Azure Machine Learning: Azure provides robust machine learning capabilities that allow manufacturers to build custom models for demand forecasting, enabling more precise inventory management.
- Tableau: By harnessing AI, Tableau transforms data visualization, allowing stakeholders to identify trends and patterns in supply chain performance, enhancing strategic planning.
AI-Driven Forecasting Techniques
Accurate forecasting is essential for maintaining supply chain efficiency. AI technologies can analyze historical data and market trends to predict future demand, allowing manufacturers to adjust their operations proactively.
Implementing AI Forecasting Solutions
- Oracle Demand Management Cloud: This tool leverages AI algorithms to generate demand forecasts based on a variety of factors, including seasonality and market trends, helping businesses align production with anticipated demand.
- SAP Integrated Business Planning: SAP’s solution integrates AI to enhance forecasting accuracy, enabling manufacturers to respond swiftly to changes in consumer behavior or market conditions.
- Kinaxis RapidResponse: This platform provides real-time analytics and scenario planning, allowing organizations to simulate different supply chain scenarios and make informed decisions based on AI-driven insights.
Real-World Applications of AI in Supply Chain Management
Several leading manufacturers have successfully implemented AI-driven analytics and forecasting tools to enhance their supply chain resilience.
Case Study: Coca-Cola
Coca-Cola has adopted AI-driven analytics to optimize its supply chain operations. By utilizing machine learning algorithms, the company has improved its demand forecasting accuracy, reducing excess inventory and minimizing stockouts.
Case Study: Unilever
Unilever employs AI to analyze consumer data and predict product demand. This approach has enabled the company to streamline its supply chain processes, resulting in significant cost savings and improved service levels.
Challenges and Considerations
While the benefits of AI in supply chain management are clear, organizations must also be aware of potential challenges. Data quality, integration with existing systems, and the need for skilled personnel to manage AI tools are critical factors that can influence the success of AI implementation.
Best Practices for Successful AI Integration
- Invest in data quality: Ensure that the data used for AI analytics is accurate and up-to-date.
- Foster a culture of collaboration: Encourage cross-departmental collaboration to ensure that insights from AI tools are effectively utilized across the organization.
- Provide training and support: Equip employees with the necessary skills to leverage AI tools effectively and maximize their potential.
Conclusion
As manufacturers navigate an increasingly complex supply chain landscape, AI-driven analytics and forecasting tools offer a pathway to enhanced resilience. By implementing these advanced technologies, organizations can gain valuable insights, improve decision-making, and ultimately achieve a more agile and responsive supply chain. Embracing AI is not just a competitive advantage; it is a necessity for survival in today’s dynamic market environment.
Keyword: AI supply chain analytics solutions