Enhancing Healthcare Chatbots with Retrieval-Augmented Generation
Topic: AI Content Tools
Industry: Healthcare
Discover how Retrieval-Augmented Generation enhances healthcare chatbots with accurate data improving patient engagement and streamlining operations in healthcare.
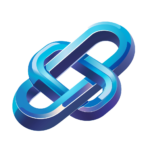
The Rise of Retrieval-Augmented Generation: Enhancing Healthcare Chatbots with Accurate Data
Understanding Retrieval-Augmented Generation (RAG)
In recent years, the integration of artificial intelligence (AI) into healthcare has transformed the way medical professionals and patients interact. One of the most promising advancements in this field is Retrieval-Augmented Generation (RAG), a technique that combines the strengths of retrieval-based systems with generative models. This hybrid approach allows chatbots to provide accurate, contextually relevant information by accessing external data sources in real-time.
The Role of AI in Healthcare Chatbots
Healthcare chatbots have become essential tools in enhancing patient engagement and streamlining administrative processes. By leveraging AI, these chatbots can deliver personalized responses, reduce wait times, and alleviate the burden on healthcare professionals. However, the effectiveness of these chatbots heavily relies on the accuracy of the data they utilize.
Benefits of RAG in Healthcare
- Enhanced Accuracy: RAG enables chatbots to access vast databases of medical information, ensuring that the responses provided are based on the latest research and clinical guidelines.
- Contextual Understanding: By utilizing retrieval mechanisms, chatbots can understand user queries better and provide more relevant answers, improving the overall user experience.
- Scalability: RAG allows healthcare organizations to scale their chatbot solutions efficiently, managing increased patient inquiries without compromising on quality.
Implementing RAG in Healthcare Chatbots
To effectively implement Retrieval-Augmented Generation in healthcare chatbots, organizations can adopt a systematic approach that includes the following steps:
1. Data Integration
Integrating reliable medical databases and knowledge repositories is crucial. This can involve partnerships with established medical institutions or leveraging existing health information systems.
2. AI Model Selection
Choosing the right AI model is vital for the success of a healthcare chatbot. Models like OpenAI’s GPT-3 or Google’s BERT can be fine-tuned to cater specifically to healthcare queries, enhancing their capability to generate accurate and relevant responses.
3. Continuous Learning
Implementing a feedback loop where chatbots learn from user interactions can significantly improve their accuracy over time. This can be achieved through machine learning techniques that analyze user feedback and adapt the chatbot’s responses accordingly.
Examples of AI-Driven Products in Healthcare
Several AI-driven tools are already making waves in the healthcare sector, demonstrating the potential of Retrieval-Augmented Generation:
1. IBM Watson Health
IBM Watson Health utilizes AI to analyze large volumes of medical data, providing healthcare professionals with evidence-based recommendations. Its integration with chatbots can enhance patient interactions by providing accurate information on treatments and medications.
2. Ada Health
Ada Health is a symptom checker that uses AI to guide users through a series of questions, ultimately providing personalized health assessments. By incorporating RAG, Ada can access up-to-date medical literature to refine its recommendations.
3. Buoy Health
Buoy Health offers an AI-driven chatbot that helps users navigate their symptoms and determine the appropriate care. With RAG, Buoy can pull data from trusted medical sources, ensuring that users receive the most accurate guidance possible.
Conclusion
The rise of Retrieval-Augmented Generation marks a significant advancement in the capabilities of healthcare chatbots. By harnessing accurate data and sophisticated AI models, healthcare organizations can enhance patient engagement, improve the quality of care, and streamline operations. As this technology continues to evolve, it holds the promise of transforming the healthcare landscape, making it more responsive and efficient for both patients and providers.
Keyword: retrieval augmented generation healthcare chatbots