Ethical AI in Healthcare R&D Navigating Challenges by 2025
Topic: AI News Tools
Industry: Research and Development
Explore the ethical considerations of AI in healthcare R&D by 2025 focusing on data privacy bias and transparency for improved patient outcomes and innovation
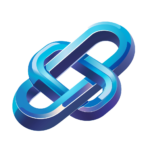
Ethical Considerations of AI in Healthcare R&D: Navigating the 2025 Landscape
Understanding the Role of AI in Healthcare R&D
The integration of artificial intelligence (AI) into healthcare research and development (R&D) is transforming the landscape of medical innovation. By 2025, we anticipate a significant expansion in the use of AI-driven tools that enhance research efficiency, improve patient outcomes, and facilitate drug discovery. However, as we embrace these advancements, it is imperative to address the ethical considerations that accompany the deployment of AI in this sensitive sector.
Key Ethical Considerations
Data Privacy and Security
One of the foremost ethical concerns in AI healthcare applications is data privacy. The sensitive nature of health data necessitates stringent measures to protect patient information. AI tools must comply with regulations such as HIPAA in the United States and GDPR in Europe, ensuring that data is anonymized and securely stored. For example, tools like IBM Watson Health leverage AI to analyze vast amounts of health data while implementing robust security protocols to safeguard privacy.
Bias and Fairness
AI systems are only as good as the data they are trained on. If the training data reflects biases, the AI outcomes can perpetuate these biases, leading to unequal healthcare delivery. It is crucial for R&D teams to utilize diverse datasets to train AI models. Tools like Google Cloud’s AutoML offer features to detect and mitigate bias, promoting fairness in AI-driven research outcomes.
Transparency and Accountability
As AI systems become more complex, understanding their decision-making processes can become challenging. Transparency in AI algorithms is essential for gaining trust among stakeholders, including researchers, healthcare providers, and patients. Platforms such as H2O.ai provide interpretable machine learning models that allow users to understand how decisions are made, fostering accountability in AI applications.
Implementing AI Tools in Healthcare R&D
AI-Driven Drug Discovery
AI can significantly accelerate the drug discovery process by analyzing biological data and predicting molecular interactions. Tools like Atomwise utilize deep learning algorithms to screen millions of compounds for potential therapeutic effects, drastically reducing the time and cost associated with traditional drug development.
Clinical Trial Optimization
AI technologies can enhance the efficiency of clinical trials by identifying suitable candidates and predicting trial outcomes. Platforms such as Deep 6 AI analyze electronic health records to match patients with clinical trials, ensuring a more targeted and effective recruitment process.
Predictive Analytics for Patient Care
AI-driven predictive analytics can improve patient care by identifying at-risk populations and facilitating early interventions. Tools like Health Catalyst leverage AI to analyze patient data, enabling healthcare providers to implement preventive measures that enhance patient outcomes and reduce costs.
Conclusion: A Balanced Approach to AI in Healthcare R&D
As we navigate the evolving landscape of AI in healthcare R&D by 2025, it is vital to maintain a balanced approach that prioritizes ethical considerations alongside technological advancements. By prioritizing data privacy, addressing bias, ensuring transparency, and implementing effective AI tools, we can harness the power of artificial intelligence to revolutionize healthcare while safeguarding the rights and well-being of patients. The future of healthcare R&D is promising, but it requires a conscientious effort to navigate the ethical complexities that come with it.
Keyword: ethical AI in healthcare R&D