Explainable AI Builds Trust in Medical Research and Healthcare
Topic: AI Health Tools
Industry: Medical research institutions
Discover how explainable AI fosters trust in medical research by enhancing transparency and interpretability in AI-driven healthcare solutions.
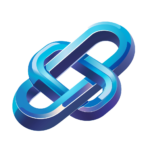
The Role of Explainable AI in Building Trust in Medical Research
Understanding Explainable AI
As artificial intelligence (AI) continues to permeate various sectors, its role in medical research is becoming increasingly significant. However, the complexity of AI algorithms often raises concerns regarding transparency and trustworthiness. Explainable AI (XAI) addresses these concerns by providing insights into how AI systems make decisions, thereby fostering trust among researchers, healthcare professionals, and patients.
The Importance of Trust in Medical Research
In the realm of medical research, trust is paramount. Researchers and clinicians must rely on data-driven insights to make informed decisions that can affect patient outcomes. When AI systems are employed, the ability to understand and interpret their recommendations is crucial. Without transparency, the adoption of AI tools may be hindered, potentially delaying advancements in healthcare.
Implementing Explainable AI in Medical Research
To effectively implement explainable AI in medical research, institutions must focus on integrating XAI principles into their AI-driven tools. This involves selecting algorithms that not only deliver accurate predictions but also provide clear explanations for their outputs. Below are some strategies and examples of AI health tools that embody these principles:
1. Algorithm Selection
Choosing the right algorithms is essential. For instance, decision trees and linear regression models are inherently more interpretable than deep learning models. Tools like IBM Watson Health utilize a combination of machine learning and natural language processing to analyze vast datasets while providing interpretable results that researchers can understand and act upon.
2. Visualization Tools
Visualization plays a crucial role in making AI decisions understandable. Tools such as SHAP (SHapley Additive exPlanations) offer visual explanations of model predictions, allowing researchers to see how different features influence outcomes. This transparency is vital for validating AI recommendations and ensuring they align with clinical knowledge.
3. Collaborative Platforms
AI-driven platforms like Google Cloud Healthcare API facilitate collaboration among researchers by providing a shared environment for data analysis. These platforms often include explainability features that allow users to explore the decision-making process behind AI models, enhancing trust and fostering a collaborative research culture.
Examples of AI-Driven Products in Medical Research
Several AI-driven products are making strides in the medical research field by incorporating explainability:
1. DeepMindās AlphaFold
AlphaFold has revolutionized protein folding prediction, a crucial aspect of understanding biological processes. Its explainability features allow researchers to comprehend the underlying mechanisms of predictions, aiding in drug discovery and development.
2. Tempus
Tempus utilizes AI to analyze clinical and molecular data to personalize cancer treatment. The platform provides interpretable insights that enable oncologists to make informed decisions based on patient-specific data, thereby enhancing trust in AI recommendations.
3. PathAI
PathAI employs machine learning to improve the accuracy of pathology diagnoses. By offering clear explanations of its predictions, PathAI helps pathologists understand the rationale behind AI-assisted diagnostics, ultimately building confidence in the technology.
Conclusion
As medical research institutions increasingly adopt AI health tools, the role of explainable AI becomes more critical. By prioritizing transparency and interpretability, researchers can build trust in AI systems, leading to more effective and reliable healthcare solutions. The integration of explainable AI not only enhances the credibility of AI-driven products but also ensures that the insights generated are actionable and aligned with clinical practices. As we move forward, fostering a culture of trust in AI will be essential for the continued advancement of medical research and patient care.
Keyword: explainable AI in medical research