Generative AI Revolutionizes Quality Control in Auto Manufacturing
Topic: AI Research Tools
Industry: Automotive
Discover how generative AI is revolutionizing quality control in automotive manufacturing by enhancing accuracy efficiency and proactive problem-solving for better products
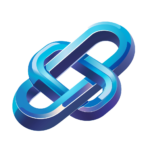
How Generative AI is Transforming Quality Control in Auto Manufacturing
The Role of Quality Control in Automotive Manufacturing
Quality control is a critical component of the automotive manufacturing process. It ensures that vehicles meet stringent safety standards, performance requirements, and customer expectations. Traditionally, quality control has relied on manual inspections and statistical sampling, which can be time-consuming and prone to human error. However, the advent of generative artificial intelligence (AI) is revolutionizing this process, enhancing accuracy, efficiency, and overall product quality.
Understanding Generative AI
Generative AI refers to a subset of artificial intelligence that can create new content or data based on existing information. In the context of automotive manufacturing, generative AI can analyze large datasets, identify patterns, and even simulate potential defects before they occur. This capability not only streamlines the quality control process but also supports proactive decision-making, allowing manufacturers to address issues before they escalate.
Implementation of Generative AI in Quality Control
Implementing generative AI in quality control requires a strategic approach. Organizations must first invest in the necessary infrastructure, including data collection systems and AI algorithms. The following are key steps to successfully integrate generative AI into quality control processes:
- Data Collection: Gather extensive data from various stages of the manufacturing process, including production metrics, inspection results, and customer feedback.
- AI Model Development: Develop predictive models using machine learning techniques to analyze the collected data and identify trends that may indicate quality issues.
- Simulation and Testing: Utilize generative AI to simulate different manufacturing scenarios and assess the potential impact on product quality.
- Continuous Improvement: Implement a feedback loop that allows the AI system to learn from new data and improve its predictive capabilities over time.
Examples of AI-Driven Tools in Quality Control
Several AI-driven tools and products are currently available to enhance quality control in automotive manufacturing. Here are some notable examples:
1. Siemens MindSphere
Siemens MindSphere is an industrial IoT platform that leverages AI and machine learning to analyze data from manufacturing processes. By providing real-time insights into production quality, it helps manufacturers identify anomalies and optimize their operations.
2. NVIDIA Clara
NVIDIA Clara is a healthcare-focused AI platform that has found applications in automotive manufacturing for quality control. It uses generative AI to analyze images and detect defects in components, ensuring that only high-quality parts make it to assembly lines.
3. IBM Watson Studio
IBM Watson Studio offers a suite of AI tools designed to facilitate data analysis and model training. In automotive manufacturing, it can be used to develop predictive maintenance models that enhance quality control by minimizing downtime and ensuring that equipment operates within specifications.
4. PTC ThingWorx
PTC ThingWorx is an IoT platform that integrates AI capabilities to monitor manufacturing processes. Its AI-driven analytics allow manufacturers to predict quality issues before they arise, thus reducing waste and improving overall product reliability.
Benefits of Generative AI in Quality Control
The benefits of incorporating generative AI into quality control processes are substantial:
- Increased Accuracy: AI systems can analyze data with a level of precision that far exceeds human capabilities, leading to more reliable quality assessments.
- Enhanced Efficiency: Automating quality control processes reduces the time required for inspections, allowing manufacturers to focus on production.
- Proactive Problem-Solving: By identifying potential defects early in the manufacturing process, generative AI enables companies to address issues before they impact production.
- Cost Savings: Improved quality control can lead to reduced rework and warranty claims, ultimately saving manufacturers significant costs.
Conclusion
As the automotive industry continues to evolve, the integration of generative AI into quality control processes represents a significant advancement. By leveraging AI-driven tools and methodologies, manufacturers can enhance product quality, improve operational efficiency, and maintain a competitive edge in a rapidly changing market. Embracing this technology is not just a trend; it is a strategic necessity for future success in automotive manufacturing.
Keyword: generative AI in automotive quality control