Generative AI Revolutionizing Demand Forecasting by 2025
Topic: AI Domain Tools
Industry: Logistics and Supply Chain
Discover how generative AI is revolutionizing demand forecasting by enhancing accuracy and adaptability for businesses in logistics and supply chain management
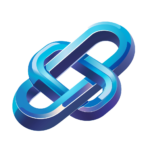
How Generative AI is Transforming Demand Forecasting: A 2025 Perspective
The Evolution of Demand Forecasting
In the ever-evolving landscape of logistics and supply chain management, demand forecasting has emerged as a critical component for operational efficiency. Traditional forecasting methods, often reliant on historical data and linear models, are increasingly inadequate in the face of dynamic market conditions. As we look towards 2025, the integration of generative AI is poised to revolutionize how businesses approach demand forecasting.
Understanding Generative AI
Generative AI refers to algorithms that can create new content or data based on existing information. Unlike traditional AI systems that primarily analyze data, generative AI can synthesize new scenarios, making it particularly valuable in demand forecasting. By leveraging vast datasets, these systems can simulate a variety of market conditions, helping businesses anticipate shifts in demand with unprecedented accuracy.
Key Benefits of Generative AI in Demand Forecasting
Enhanced Accuracy
One of the most significant advantages of generative AI is its ability to improve forecasting accuracy. By analyzing complex patterns and correlations within data, generative AI can identify trends that may not be immediately apparent through conventional methods. This leads to more reliable forecasts, allowing companies to optimize inventory levels and reduce costs.
Real-Time Adaptability
Market conditions can change rapidly, and businesses must adapt accordingly. Generative AI tools can process real-time data inputs, enabling companies to adjust their forecasts on-the-fly. This adaptability is crucial for maintaining competitiveness in a fast-paced environment.
Scenario Simulation
Generative AI can create multiple demand scenarios based on varying factors such as seasonality, economic shifts, or consumer behavior changes. This capability allows businesses to prepare for different outcomes and devise strategies to mitigate risks associated with demand fluctuations.
Implementing Generative AI in Demand Forecasting
To harness the power of generative AI in demand forecasting, companies must consider the following steps:
1. Data Integration
Successful implementation begins with integrating diverse data sources, including historical sales data, market trends, and external factors such as economic indicators. Tools like Tableau and Microsoft Power BI can help visualize and combine these datasets for analysis.
2. Choosing the Right Tools
Several AI-driven tools are available to facilitate generative AI applications in demand forecasting:
- IBM Watson Studio: This platform provides advanced analytics capabilities, allowing organizations to build and train generative AI models tailored to their specific forecasting needs.
- Google Cloud AI: Google’s suite of AI tools can analyze vast amounts of data and generate predictive models, making it easier for businesses to forecast demand accurately.
- Microsoft Azure Machine Learning: Azure offers a comprehensive set of tools for developing machine learning models, including those that leverage generative AI for demand forecasting.
3. Continuous Learning and Improvement
Generative AI systems thrive on continuous learning. Companies should regularly update their models with new data to enhance accuracy over time. This iterative process ensures that forecasts remain relevant and responsive to market changes.
Case Studies of Generative AI in Action
Example 1: Retail Industry
A leading retail chain implemented a generative AI solution to enhance its demand forecasting capabilities. By integrating real-time sales data with external factors such as weather patterns and social media trends, the company achieved a 20% reduction in stockouts and improved inventory turnover rates.
Example 2: Consumer Electronics
A consumer electronics manufacturer utilized generative AI to simulate various demand scenarios during product launches. This approach allowed the company to allocate resources more effectively, resulting in a 15% increase in sales during peak periods.
Conclusion
As we approach 2025, generative AI is set to transform demand forecasting in logistics and supply chain management. By enhancing accuracy, enabling real-time adaptability, and facilitating scenario simulation, businesses can better navigate the complexities of modern markets. The implementation of AI-driven tools will not only streamline operations but also drive significant competitive advantages in an increasingly data-driven world.
Keyword: generative AI demand forecasting