Generative AI Revolutionizing Financial Forecasting and Planning
Topic: AI Analytics Tools
Industry: Finance and Banking
Discover how generative AI is transforming financial forecasting and planning with enhanced accuracy real-time insights and cost efficiency for financial institutions
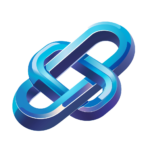
How Generative AI is Transforming Financial Forecasting and Planning
The Evolution of Financial Forecasting
Financial forecasting and planning have traditionally relied on historical data analysis, expert judgment, and basic statistical methods. However, the rapid advancement of technology, particularly in artificial intelligence (AI), is reshaping these processes. Generative AI, a subset of artificial intelligence, is emerging as a powerful tool that enhances forecasting accuracy, optimizes planning, and drives strategic decision-making in finance and banking.
Understanding Generative AI in Finance
Generative AI refers to algorithms that can generate new content or data based on existing information. In the context of financial forecasting, these models analyze vast datasets to identify patterns, simulate scenarios, and produce predictive insights. By leveraging generative AI, financial institutions can move beyond traditional methods, allowing for more dynamic and responsive planning.
Key Benefits of Generative AI in Financial Forecasting
- Enhanced Accuracy: Generative AI models can process large volumes of data with speed and precision, leading to more accurate forecasts.
- Scenario Simulation: These tools can simulate various economic scenarios, helping businesses understand potential risks and opportunities.
- Real-time Insights: Generative AI enables continuous analysis, providing real-time insights that support agile decision-making.
- Cost Efficiency: By automating data analysis and forecasting processes, organizations can reduce operational costs and allocate resources more effectively.
Implementing Generative AI in Financial Institutions
To harness the power of generative AI, financial institutions must adopt a strategic approach. Implementation involves several key steps:
1. Data Integration
Successful AI implementation starts with robust data integration. Financial institutions must consolidate data from various sources, including transactional data, market trends, and economic indicators. This comprehensive dataset serves as the foundation for AI models.
2. Choosing the Right Tools
Selecting appropriate AI-driven tools is crucial. Here are some notable examples:
- IBM Watson: Known for its powerful analytics capabilities, IBM Watson can analyze unstructured data and generate insights, making it a valuable tool for financial forecasting.
- Tableau: While primarily a data visualization tool, Tableau’s AI features allow users to create predictive models that enhance forecasting accuracy.
- Microsoft Azure Machine Learning: This platform provides a suite of tools for building, training, and deploying AI models, allowing financial institutions to create customized forecasting solutions.
- Alteryx: Alteryx combines data preparation, analytics, and machine learning, enabling finance teams to automate forecasting processes and gain actionable insights.
3. Training and Development
Investing in training and development is essential for staff to effectively utilize AI tools. This includes understanding how to interpret AI-generated insights and integrate them into strategic planning.
4. Continuous Monitoring and Improvement
AI models require ongoing monitoring to ensure accuracy and relevance. Financial institutions should establish protocols for regularly updating models based on new data and changing market conditions.
Case Studies: Success Stories in Generative AI Implementation
Several financial institutions have successfully implemented generative AI to enhance their forecasting and planning capabilities:
1. JPMorgan Chase
JPMorgan Chase has integrated AI-driven analytics tools to enhance its forecasting accuracy. By utilizing machine learning algorithms to analyze market data, the bank has improved its ability to predict economic trends and adjust its strategies accordingly.
2. Goldman Sachs
Goldman Sachs employs generative AI to simulate various market scenarios, enabling its analysts to make informed decisions based on potential risks and opportunities. This proactive approach has strengthened their strategic planning processes.
Conclusion
Generative AI is revolutionizing financial forecasting and planning by providing enhanced accuracy, real-time insights, and cost efficiency. As financial institutions continue to adopt AI-driven tools, they will gain a competitive edge in an increasingly complex market. By integrating generative AI into their forecasting processes, organizations can not only navigate uncertainties but also identify new growth opportunities, ultimately driving their success in the financial landscape.
Keyword: Generative AI financial forecasting