Generative AI Transforming Energy Forecasting and Demand Response
Topic: AI Collaboration Tools
Industry: Energy and Utilities
Discover how generative AI transforms energy forecasting and demand response enhancing accuracy and efficiency for utilities and energy management solutions
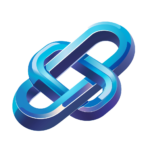
The Impact of Generative AI on Energy Forecasting and Demand Response
Introduction to Generative AI in Energy Sector
Generative AI is revolutionizing various industries, and the energy sector is no exception. The integration of AI collaboration tools in energy and utilities is enhancing the accuracy of energy forecasting and improving demand response strategies. By leveraging generative AI, organizations can analyze vast datasets, predict energy consumption patterns, and optimize resource allocation.
Energy Forecasting: Transforming Predictions
Energy forecasting involves predicting future energy demand based on historical data, weather patterns, and other influencing factors. Traditional methods often struggle to account for the complexities of real-time data. However, generative AI tools can enhance forecasting accuracy significantly.
AI-Driven Forecasting Tools
Several AI-driven products are emerging as leaders in the energy forecasting space:
- AutoGrid: This platform utilizes machine learning algorithms to analyze historical energy consumption data, enabling utilities to forecast demand with greater precision.
- Uplight: Uplight offers predictive analytics tools that help utilities understand customer behavior and adjust forecasts accordingly, ensuring a more reliable energy supply.
- IBM Watson: IBM’s AI solutions provide advanced analytics capabilities that can integrate weather forecasts, economic indicators, and historical usage data to improve demand predictions.
Demand Response: Enhancing Energy Management
Demand response (DR) programs are essential for balancing energy supply and demand, especially during peak usage times. Generative AI can optimize these programs by analyzing real-time data and predicting when and where demand will spike.
AI Tools for Demand Response
Several AI collaboration tools are specifically designed to enhance demand response initiatives:
- EnergyHub: This platform allows utilities to manage demand response programs effectively by leveraging AI to analyze customer usage patterns and engage them in energy-saving initiatives.
- Enel X: Enel X utilizes AI to create dynamic demand response solutions that adjust in real-time based on grid conditions and customer participation.
- Siemens Demand Response Management: This tool uses AI algorithms to optimize demand response strategies, ensuring that energy resources are allocated efficiently during peak periods.
Implementation of Generative AI in Energy and Utilities
Implementing generative AI in energy forecasting and demand response requires a strategic approach. Organizations should consider the following steps:
1. Data Integration
Successful AI implementation begins with integrating diverse data sources, including historical consumption data, weather forecasts, and customer behavior analytics. This comprehensive dataset enables more accurate predictions.
2. Selecting the Right Tools
Choosing the appropriate generative AI tools is crucial. Organizations should evaluate various AI-driven products based on their specific needs, scalability, and integration capabilities with existing systems.
3. Training and Development
Investing in training for staff on how to use AI tools effectively is essential. This ensures that teams can leverage the full potential of generative AI in their forecasting and demand response efforts.
4. Continuous Monitoring and Improvement
AI models require continuous monitoring and refinement to adapt to changing conditions. Regularly assessing the performance of AI tools will help organizations stay ahead of trends and improve their forecasting accuracy.
Conclusion
The impact of generative AI on energy forecasting and demand response is profound. By implementing AI collaboration tools, energy and utility companies can enhance their predictive capabilities, streamline operations, and respond more effectively to consumer needs. As the energy landscape continues to evolve, embracing these technologies will be key to achieving sustainability and efficiency in energy management.
Keyword: Generative AI in energy forecasting