Harnessing NLP for Enhanced Biomedical Literature Analysis
Topic: AI Developer Tools
Industry: Pharmaceuticals and Biotechnology
Discover how Natural Language Processing enhances biomedical literature analysis in pharmaceuticals and biotechnology to streamline research and extract valuable insights
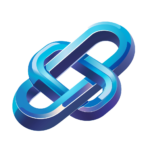
Harnessing Natural Language Processing for Biomedical Literature Analysis
In the rapidly evolving fields of pharmaceuticals and biotechnology, the volume of biomedical literature is growing exponentially. This surge presents both opportunities and challenges for researchers and developers. To navigate this complex landscape, organizations are increasingly turning to artificial intelligence (AI) and, more specifically, Natural Language Processing (NLP) to enhance their literature analysis capabilities.
The Role of Natural Language Processing in Biomedical Research
NLP is a branch of AI that focuses on the interaction between computers and human language. It enables machines to understand, interpret, and generate human language in a valuable way. In the context of biomedical literature, NLP can be utilized to extract relevant information, identify trends, and facilitate knowledge discovery from vast datasets.
Key Applications of NLP in Biomedical Literature Analysis
Several key applications of NLP in the analysis of biomedical literature include:
- Information Extraction: NLP algorithms can identify and extract specific data points from research papers, such as drug names, disease classifications, and clinical trial results.
- Sentiment Analysis: By analyzing the sentiment of published studies, researchers can gauge the overall consensus on particular treatments or findings.
- Topic Modeling: NLP can be employed to categorize literature into topics, helping researchers quickly identify relevant studies in a specific area of interest.
- Literature Summarization: Automated summarization tools can condense lengthy articles into concise abstracts, saving time for researchers who need to stay informed.
Implementing AI-Driven Tools in Biomedical Literature Analysis
To effectively harness the power of NLP, pharmaceutical and biotechnology companies can leverage various AI-driven tools designed for literature analysis. Here are a few notable examples:
1. IBM Watson Discovery
IBM Watson Discovery utilizes NLP to analyze unstructured data, including scientific literature. Its powerful search capabilities allow researchers to uncover insights from millions of documents, enabling them to stay ahead of the curve in drug development and therapeutic research.
2. Elsevier’s PharmaPendium
PharmaPendium is a comprehensive resource that uses NLP to extract and present FDA drug approval documents. By providing detailed information on drug indications, side effects, and clinical trial results, it empowers researchers to make informed decisions based on the latest available data.
3. BioBERT
BioBERT is a pre-trained biomedical language representation model based on BERT (Bidirectional Encoder Representations from Transformers). It excels in various NLP tasks, such as named entity recognition and relation extraction, making it an invaluable tool for researchers looking to automate literature reviews and data extraction.
4. PubMed Central (PMC) Text Mining Tools
PMC offers a range of text mining tools that leverage NLP to facilitate access to biomedical literature. These tools allow researchers to perform bulk downloads of articles and apply custom algorithms for data extraction, significantly streamlining the research process.
Challenges and Considerations
While the potential of NLP in biomedical literature analysis is immense, organizations must also consider several challenges:
- Data Quality: The effectiveness of NLP models heavily relies on the quality of the data they are trained on. Ensuring that the underlying datasets are accurate and representative is crucial.
- Domain-Specific Language: Biomedical literature often contains specialized terminology and jargon that can pose challenges for NLP models. Tailoring models to understand this language is essential for effective analysis.
- Ethical Considerations: As with any AI application, ethical considerations around data privacy and the potential for bias must be addressed to maintain trust in AI-driven solutions.
Conclusion
As the pharmaceutical and biotechnology sectors continue to expand, harnessing Natural Language Processing for biomedical literature analysis is becoming increasingly vital. By implementing AI-driven tools, organizations can streamline their research processes, extract valuable insights, and ultimately enhance their contributions to the field. Embracing these technologies not only accelerates innovation but also positions companies to thrive in an increasingly competitive landscape.
Keyword: biomedical literature analysis tools