Leverage Machine Learning to Enhance Soil Health for Farmers
Topic: AI Business Tools
Industry: Agriculture
Discover how machine learning enhances soil health analysis for farmers Optimize crop yields and sustainability with AI-driven tools and insights
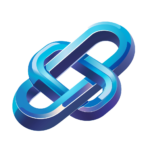
Leveraging Machine Learning for Soil Health Analysis: A Guide for Farmers
Understanding Soil Health and Its Importance
Soil health is a critical determinant of agricultural productivity and sustainability. Healthy soil supports plant growth, enhances water retention, and promotes biodiversity. Farmers are increasingly recognizing the need to monitor and improve soil health to optimize crop yields and mitigate environmental impacts. This is where machine learning (ML) and artificial intelligence (AI) come into play, offering innovative tools to analyze soil health efficiently and effectively.
The Role of Machine Learning in Soil Health Analysis
Machine learning, a subset of artificial intelligence, involves the use of algorithms that learn from data to make predictions or decisions without being explicitly programmed. In the context of soil health, ML can analyze vast amounts of data collected from various sources, providing insights that can help farmers make informed decisions.
Data Collection Methods
To leverage machine learning for soil health analysis, farmers need to collect data through various methods, including:
- Soil Sampling: Regular sampling allows for the collection of physical and chemical properties of the soil.
- Remote Sensing: Satellite imagery and drones can capture data on soil moisture, temperature, and vegetation health.
- Sensor Technology: Soil sensors can provide real-time data on moisture levels, pH, and nutrient content.
Implementing Machine Learning Solutions
Once data is collected, machine learning algorithms can be applied to analyze the information. Here are some ways AI can be implemented in soil health analysis:
- Predictive Analytics: ML can predict soil health trends by analyzing historical data, helping farmers anticipate issues before they arise.
- Soil Classification: Algorithms can classify soil types based on their properties, allowing for tailored management practices.
- Yield Prediction: By correlating soil health data with crop yields, farmers can optimize their planting strategies.
Examples of AI-Driven Tools for Soil Health Analysis
Several AI-driven products and tools are available to assist farmers in analyzing soil health:
1. SoilOptix
SoilOptix offers a soil mapping solution that utilizes advanced algorithms to analyze soil properties at a granular level. This tool provides insights into nutrient distribution and helps farmers make data-driven decisions regarding fertilization and crop rotation.
2. AgriWebb
AgriWebb is a farm management software that incorporates machine learning to analyze soil health data. It provides farmers with actionable insights by integrating data from soil tests, weather patterns, and crop performance.
3. CropX
CropX combines soil sensors and machine learning to provide real-time soil health analysis. The platform offers recommendations for irrigation and fertilization based on the current state of the soil, helping farmers optimize resource use.
4. IBM Watson Decision Platform for Agriculture
IBM’s Watson Decision Platform integrates AI, IoT, and blockchain to provide comprehensive insights into soil health. By analyzing data from various sources, it helps farmers improve productivity and sustainability through informed decision-making.
Conclusion
As agriculture faces increasing challenges from climate change and population growth, leveraging machine learning for soil health analysis presents a promising solution. By utilizing AI-driven tools, farmers can gain valuable insights into their soil, leading to improved crop yields, enhanced sustainability, and better resource management. Embracing these technologies not only benefits individual farmers but also contributes to the broader goal of sustainable agriculture.
Keyword: machine learning soil health analysis