Machine Learning Anomaly Detection in Industrial IoT Networks
Topic: AI Security Tools
Industry: Manufacturing
Discover how machine learning enhances anomaly detection in Industrial IoT networks to improve security and operational efficiency in manufacturing environments
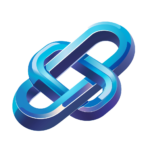
Machine Learning for Anomaly Detection in Industrial IoT Networks
Understanding the Importance of Anomaly Detection
In the rapidly evolving landscape of manufacturing, the integration of Industrial Internet of Things (IIoT) devices has revolutionized operational efficiency. However, this technological advancement also introduces significant security vulnerabilities. Anomaly detection, powered by machine learning (ML), is essential for identifying irregular patterns that may indicate potential threats or failures within these networks. By leveraging AI security tools, manufacturers can enhance their resilience against cyber threats while optimizing their operational processes.
How Machine Learning Enhances Anomaly Detection
Machine learning algorithms analyze vast amounts of data generated by IIoT devices to identify trends and detect anomalies in real-time. These algorithms can learn from historical data, making them adept at recognizing what constitutes normal behavior within a network. When deviations from this norm occur, the system can alert operators, enabling swift action to mitigate risks.
Key Techniques in Machine Learning for Anomaly Detection
- Supervised Learning: This method involves training models on labeled datasets, where the algorithm learns to distinguish between normal and anomalous behavior based on predefined labels.
- Unsupervised Learning: In scenarios where labeled data is scarce, unsupervised learning techniques can identify anomalies based solely on the inherent structure of the data.
- Reinforcement Learning: This technique allows systems to learn optimal actions through trial and error, making it suitable for dynamic environments where threats can evolve.
Implementing AI Security Tools in Manufacturing
To effectively deploy machine learning for anomaly detection, manufacturers can utilize a variety of AI-driven products and tools. Below are some notable examples:
1. IBM Watson IoT Platform
The IBM Watson IoT Platform integrates AI capabilities to provide real-time analytics and anomaly detection for IIoT networks. By analyzing sensor data, it can identify deviations and predict equipment failures, allowing for proactive maintenance and reducing downtime.
2. Microsoft Azure IoT
Microsoft’s Azure IoT suite offers advanced analytics and machine learning capabilities for anomaly detection. With its built-in algorithms, manufacturers can monitor devices and receive alerts for unusual patterns, enhancing security and operational efficiency.
3. Google Cloud AI
Google Cloud AI provides tools such as AutoML and BigQuery ML, which enable manufacturers to build custom machine learning models for anomaly detection. These tools allow for the analysis of large datasets, facilitating the identification of anomalies that could indicate security breaches or operational failures.
4. Siemens MindSphere
Siemens MindSphere is an industrial IoT platform that employs machine learning algorithms to analyze data from connected devices. It offers anomaly detection features that help manufacturers optimize their production processes and enhance security measures.
Challenges and Considerations
While the benefits of machine learning for anomaly detection are significant, manufacturers must also be aware of potential challenges. Data quality and availability are critical; without accurate and comprehensive datasets, the effectiveness of machine learning models may be compromised. Additionally, the complexity of integrating these AI tools into existing systems can pose hurdles, requiring careful planning and execution.
Conclusion
As the manufacturing sector continues to embrace IIoT technologies, the need for robust security measures becomes increasingly vital. Machine learning for anomaly detection presents a powerful solution to safeguard industrial networks against potential threats. By implementing AI-driven tools, manufacturers can enhance their operational resilience, ensuring a secure and efficient production environment.
Keyword: industrial IoT anomaly detection