Machine Learning Enhances Incident Response in Cybersecurity
Topic: AI Other Tools
Industry: Cybersecurity
Discover how machine learning transforms incident response in cybersecurity by enhancing speed accuracy and automation for effective threat management.
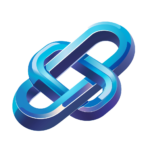
The Role of Machine Learning in Automating Incident Response
Understanding Incident Response in Cybersecurity
Incident response is a critical component of any cybersecurity strategy. It involves the processes and procedures organizations implement to identify, manage, and mitigate cybersecurity incidents. In an era where cyber threats are becoming more sophisticated and frequent, the need for efficient incident response mechanisms has never been more pressing. This is where machine learning (ML) and artificial intelligence (AI) come into play, revolutionizing the way organizations manage and respond to incidents.
Machine Learning: A Game Changer for Incident Response
Machine learning, a subset of artificial intelligence, enables systems to learn from data, identify patterns, and make decisions with minimal human intervention. In the context of incident response, ML can significantly enhance the speed and effectiveness of detecting and responding to security threats.
Key Benefits of Machine Learning in Incident Response
- Speed: ML algorithms can analyze vast amounts of data in real-time, allowing for quicker identification of potential threats.
- Accuracy: By learning from historical data, ML models can improve their predictive capabilities, reducing false positives and negatives in threat detection.
- Automation: Routine tasks can be automated, freeing up cybersecurity professionals to focus on more complex issues.
Implementing AI and Machine Learning in Incident Response
Organizations looking to implement AI and machine learning in their incident response strategies can consider several approaches and tools. Here are some examples of AI-driven products that can be utilized:
1. SIEM Solutions with AI Capabilities
Security Information and Event Management (SIEM) solutions are essential for incident response. Modern SIEM tools, such as Splunk and IBM QRadar, have integrated machine learning capabilities that help in detecting anomalies and potential threats in real-time. These tools can analyze log data and correlate events to identify patterns indicative of security incidents.
2. Automated Threat Intelligence Platforms
Platforms like Recorded Future and ThreatConnect leverage machine learning to aggregate and analyze threat intelligence. By automating the collection and analysis of threat data, these tools provide actionable insights that can inform incident response strategies and improve overall security posture.
3. Endpoint Detection and Response (EDR) Tools
EDR solutions such as CrowdStrike and Carbon Black utilize machine learning algorithms to monitor endpoint activities and detect suspicious behavior. These tools can automatically respond to threats by isolating affected systems or deploying remediation measures, thereby minimizing the impact of security incidents.
4. Security Automation and Orchestration
Security orchestration platforms like Palo Alto Networks Cortex XSOAR and ServiceNow Security Operations enable organizations to automate incident response workflows. By integrating machine learning, these platforms can prioritize incidents, suggest remediation actions, and streamline communication among security teams.
Challenges and Considerations
While the benefits of incorporating machine learning into incident response are clear, organizations must also be aware of potential challenges. Data quality is paramount; ML models require high-quality, relevant data to function effectively. Additionally, organizations should ensure they have the necessary expertise to implement and manage these advanced tools. Continuous monitoring and model training are crucial to maintain accuracy and effectiveness.
Conclusion
The integration of machine learning into incident response processes represents a significant advancement in the field of cybersecurity. By leveraging AI-driven tools, organizations can enhance their ability to detect, respond to, and recover from cyber incidents more efficiently and effectively. As the threat landscape continues to evolve, embracing these technologies will be essential for organizations looking to protect their assets and maintain operational resilience.
Keyword: machine learning incident response automation