Machine Learning Enhances Renewable Energy Output Predictions
Topic: AI Other Tools
Industry: Energy and Utilities
Discover how machine learning enhances renewable energy output predictions improving accuracy and efficiency for a sustainable energy future
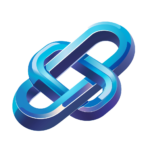
The Role of Machine Learning in Predicting Renewable Energy Output
The increasing reliance on renewable energy sources necessitates accurate predictions of energy output to enhance grid stability and optimize resource allocation. Machine learning (ML), a subset of artificial intelligence (AI), has emerged as a vital tool in this domain, enabling energy providers to harness data-driven insights for improved decision-making.
Understanding Machine Learning in Energy Forecasting
Machine learning algorithms analyze vast datasets to identify patterns and trends that may not be immediately apparent to human analysts. By leveraging historical data on weather conditions, energy consumption, and production metrics, ML models can forecast renewable energy output with remarkable precision.
Key Benefits of Machine Learning in Renewable Energy
- Enhanced Accuracy: ML models continuously learn from new data, refining their predictions over time and improving accuracy in forecasting energy output.
- Real-Time Analysis: With the ability to process data in real-time, machine learning can provide immediate insights, allowing utilities to respond swiftly to changing conditions.
- Cost Efficiency: By optimizing energy production and reducing waste, ML contributes to overall cost savings for energy providers.
Implementation of AI in Renewable Energy
Integrating AI-driven tools into renewable energy operations can significantly enhance forecasting capabilities. Here are several specific tools and products that illustrate the practical application of machine learning in this sector:
1. IBM Watson Studio
IBM Watson Studio offers a suite of machine learning tools designed for data scientists and engineers. Its capabilities in predictive analytics allow energy companies to model and forecast renewable energy output based on historical weather data and production metrics. By using Watson Studio, utilities can gain insights into potential energy generation from solar or wind sources, facilitating better grid management.
2. Google Cloud AI
Google Cloud AI provides advanced machine learning capabilities that can be utilized for energy forecasting. With tools like AutoML, energy providers can create custom models tailored to their specific needs. For example, a solar energy provider can use Google Cloud AI to predict daily energy output based on cloud cover and temperature data, allowing for better integration into the energy grid.
3. Siemens’ Predictive Analytics Solutions
Siemens has developed predictive analytics solutions that leverage machine learning to optimize energy production. Their software analyzes data from wind turbines and solar panels to forecast output accurately, enabling utilities to adjust their operations proactively. This not only maximizes energy efficiency but also minimizes downtime and maintenance costs.
4. ENEL’s Advanced Forecasting System
ENEL, a leading energy company, employs machine learning algorithms to predict renewable energy output across its global operations. By utilizing historical performance data and real-time weather information, ENEL’s advanced forecasting system enhances their ability to manage energy distribution effectively. This system plays a crucial role in integrating renewable sources into the energy mix, ensuring a reliable supply.
The Future of Machine Learning in Renewable Energy
As technology continues to evolve, the role of machine learning in predicting renewable energy output is expected to expand further. The integration of more sophisticated algorithms, coupled with the increasing availability of big data, will enable even greater accuracy in forecasting. Furthermore, as energy markets become more competitive, the ability to predict energy production will serve as a critical differentiator for companies striving to optimize their operations.
Conclusion
Machine learning is revolutionizing the way energy providers predict renewable energy output. By implementing AI-driven tools and solutions, companies can enhance their forecasting capabilities, improve operational efficiency, and contribute to a more sustainable energy future. As the industry continues to embrace these technologies, the potential for innovation and growth in the renewable energy sector remains vast.
Keyword: machine learning renewable energy forecasting