Machine Learning for Demand Forecasting in Manufacturing
Topic: AI Developer Tools
Industry: Manufacturing
Discover how machine learning models enhance demand forecasting in manufacturing improve accuracy reduce costs and boost customer satisfaction for competitive advantage
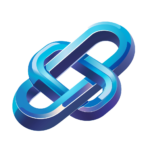
Machine Learning Models for Demand Forecasting: Tools Manufacturers Can’t Ignore
Understanding Demand Forecasting in Manufacturing
Demand forecasting is a critical component of supply chain management in the manufacturing sector. It involves predicting future customer demand for products, enabling manufacturers to optimize production schedules, manage inventory levels, and enhance customer satisfaction. Traditional forecasting methods often rely on historical sales data and market trends, but they can be limited in their accuracy and responsiveness to real-time changes. This is where machine learning (ML) models come into play, offering advanced capabilities that can significantly improve forecasting outcomes.The Role of Machine Learning in Demand Forecasting
Machine learning models leverage vast amounts of data and sophisticated algorithms to identify patterns and trends that may not be apparent through conventional analysis. By analyzing historical data, market conditions, and even external factors such as economic indicators or social media sentiment, these models can generate more accurate demand forecasts.Key Benefits of Using Machine Learning for Demand Forecasting
1. Enhanced Accuracy: ML models can process and analyze data at a scale and speed that human analysts cannot match, leading to more precise forecasts. 2. Real-Time Adaptation: Machine learning algorithms can continuously learn from new data, allowing them to adapt to changing market conditions in real time. 3. Integration of Diverse Data Sources: ML can incorporate data from various sources, including IoT devices, CRM systems, and external market data, providing a holistic view of demand factors. 4. Reduced Costs: Improved forecasting accuracy can lead to lower inventory holding costs and reduced waste, ultimately enhancing profitability.Implementing Machine Learning Models in Manufacturing
To effectively implement machine learning for demand forecasting, manufacturers should consider the following steps:1. Data Collection and Preparation
The first step involves gathering relevant data from various sources. This includes historical sales data, production data, market trends, and external factors. Data must be cleaned and pre-processed to ensure its quality and usability for machine learning models.2. Choosing the Right Machine Learning Tools
Several AI-driven tools and platforms can assist manufacturers in building and deploying machine learning models. Here are a few notable examples: – Microsoft Azure Machine Learning: This cloud-based platform provides a suite of tools for building, training, and deploying machine learning models. It supports a variety of algorithms and can integrate with other Azure services for data storage and processing. – Google Cloud AI: Google’s AI tools offer powerful machine learning capabilities, including AutoML, which allows users to build custom models without extensive programming knowledge. Its integration with BigQuery enables efficient data analysis and management. – IBM Watson Studio: IBM’s platform provides a collaborative environment for data scientists and engineers to develop machine learning models. It includes built-in tools for data preparation, model training, and deployment. – Amazon SageMaker: This service allows developers to build, train, and deploy machine learning models quickly. It provides a range of built-in algorithms and supports custom model development.3. Model Training and Validation
Once the appropriate tools are selected, manufacturers can begin training their models using historical data. It’s crucial to validate the models using separate datasets to ensure their accuracy and reliability in predicting future demand.4. Deployment and Monitoring
After successful training and validation, the models can be deployed into production systems. Continuous monitoring is essential to assess model performance and make adjustments as necessary, ensuring that forecasts remain accurate over time.Case Studies: Successful Implementations
Several manufacturers have successfully implemented machine learning for demand forecasting, yielding impressive results: – Unilever: By utilizing machine learning algorithms, Unilever improved its demand forecasting accuracy by 25%. This enhancement led to better inventory management and reduced stockouts. – Procter & Gamble: P&G adopted advanced analytics and machine learning to optimize its supply chain processes. The company reported a significant reduction in inventory costs while maintaining high service levels.Conclusion
In an increasingly competitive manufacturing landscape, leveraging machine learning models for demand forecasting is no longer optional; it has become a necessity. The ability to accurately predict demand can lead to enhanced operational efficiency, cost savings, and improved customer satisfaction. By adopting AI-driven tools and integrating them into their forecasting processes, manufacturers can position themselves for success in a rapidly evolving market. Embracing these technologies will not only streamline operations but also pave the way for innovation and growth in the manufacturing sector.Keyword: machine learning demand forecasting tools