Machine Learning for Predicting Furniture Trends and Consumer Needs
Topic: AI Design Tools
Industry: Furniture Design
Discover how machine learning is revolutionizing furniture design by predicting trends and enhancing customization to meet evolving consumer preferences.
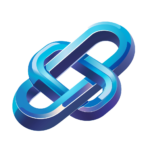
Machine Learning in Furniture Trend Prediction: Staying Ahead of Consumer Preferences
Understanding the Role of Machine Learning in Furniture Design
In the rapidly evolving world of furniture design, staying ahead of consumer preferences is crucial for success. Machine learning (ML), a subset of artificial intelligence (AI), is increasingly being leveraged to predict trends and inform design decisions. By analyzing vast amounts of data, ML algorithms can identify patterns and forecast shifts in consumer tastes, enabling designers and manufacturers to create products that resonate with their target audience.Implementing AI in Furniture Design
The integration of AI-driven tools in the furniture design process can significantly enhance efficiency and creativity. Here are several ways in which machine learning can be implemented:1. Data-Driven Insights
Machine learning algorithms can analyze consumer behavior data from various sources, including social media, e-commerce platforms, and market research reports. By processing this information, designers can gain insights into emerging trends, preferred materials, and color palettes. For instance, tools like Google Trends can provide real-time data on search queries related to furniture styles, helping designers stay informed about what consumers are currently interested in.2. Predictive Analytics
Predictive analytics powered by machine learning can forecast future trends based on historical data. By examining past sales data, customer feedback, and design trends, AI tools can generate predictions about which styles or features are likely to gain popularity. For example, platforms like Trendalytics utilize AI to offer predictive insights into consumer preferences, enabling furniture companies to make informed decisions about product development.3. Customization and Personalization
AI-driven design tools can also facilitate customization, allowing consumers to personalize their furniture choices. By analyzing user preferences and past purchases, these tools can suggest tailored designs that align with individual tastes. Companies like IKEA have begun to implement AI in their design processes, offering customers the ability to visualize how different furniture pieces will look in their homes through augmented reality applications.Examples of AI-Driven Tools in Furniture Design
Several innovative tools are currently transforming the furniture design landscape through machine learning:1. Modsy
Modsy is an online interior design service that uses AI to create 3D renderings of rooms based on user inputs. By analyzing user preferences and existing furniture, Modsy provides personalized design recommendations, helping consumers visualize their ideal space before making a purchase.2. Houzz
Houzz is a platform that connects homeowners with design professionals. Its AI-driven features allow users to explore design ideas and receive tailored recommendations based on their style preferences and project needs. By leveraging user data, Houzz can suggest furniture and decor that align with current trends.3. Autodesk Generative Design
Autodesk’s generative design software uses machine learning algorithms to explore a multitude of design options based on user-defined constraints. This tool enables furniture designers to create innovative and efficient designs that meet specific criteria while taking into account material properties and manufacturing methods.Conclusion: The Future of Furniture Design with AI
As consumer preferences continue to evolve, the integration of machine learning in furniture trend prediction will become increasingly vital. By harnessing the power of AI-driven tools, designers and manufacturers can stay ahead of the curve, ensuring that their offerings align with market demands. Embracing these technologies not only enhances the design process but also fosters a deeper understanding of consumer behavior, ultimately leading to more successful products in the competitive furniture market.Keyword: machine learning furniture design trends