Machine Learning for Real-Time Pest and Disease Detection
Topic: AI Data Tools
Industry: Agriculture
Discover how machine learning enhances pest and disease detection in agriculture enabling real-time crop protection and optimizing resource use for farmers
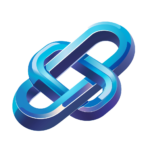
Machine Learning for Pest and Disease Detection: Protecting Crops in Real-Time
Introduction to AI in Agriculture
The agricultural sector faces numerous challenges, from climate change to resource scarcity, but one of the most pressing issues is pest and disease management. Traditional methods of monitoring crops can be labor-intensive and often lack the precision needed for timely interventions. Fortunately, advancements in artificial intelligence (AI) and machine learning (ML) are transforming how farmers detect and respond to these threats, enabling real-time protection of crops.
Understanding Machine Learning in Pest and Disease Detection
Machine learning, a subset of AI, involves the development of algorithms that allow computers to learn from and make predictions based on data. In the context of agriculture, ML can analyze vast amounts of data from various sources, including satellite imagery, weather patterns, and soil conditions, to identify potential pest and disease outbreaks before they escalate.
Key Benefits of ML in Agriculture
- Real-Time Monitoring: ML algorithms can process data in real-time, providing immediate insights into crop health.
- Increased Accuracy: By analyzing historical data and current conditions, AI can improve the accuracy of pest and disease predictions.
- Resource Optimization: Targeted interventions reduce waste and optimize the use of pesticides and fertilizers.
Implementation of AI Tools in Pest and Disease Detection
Several AI-driven tools and products are currently available to assist farmers in pest and disease detection. These tools leverage machine learning algorithms to provide actionable insights, enhancing decision-making processes in agriculture.
1. Crop Health Monitoring Platforms
Platforms like CropX utilize soil sensor data combined with weather information to provide farmers with real-time insights into crop health. By integrating machine learning, CropX can detect anomalies that may indicate pest infestations or disease outbreaks, allowing for timely interventions.
2. Drone Technology
Drones equipped with multispectral cameras, such as those offered by DJI, can capture high-resolution images of fields. These images are analyzed using machine learning algorithms to identify stress patterns in crops that may suggest pest or disease issues. This aerial perspective allows farmers to cover large areas quickly and efficiently.
3. Mobile Applications
Applications like Plantix allow users to take pictures of plants and receive instant diagnoses of potential diseases or pest infestations. The app uses machine learning to compare the images against a vast database of plant diseases, providing farmers with immediate feedback and recommendations for treatment.
4. Predictive Analytics Tools
Tools such as AgriMetriX employ predictive analytics to forecast pest outbreaks based on environmental conditions and historical data. By utilizing machine learning models, these tools can help farmers prepare and implement preventative measures before an outbreak occurs.
Challenges and Considerations
While the benefits of machine learning in pest and disease detection are significant, there are challenges to consider. Data quality and availability are critical; without accurate and comprehensive data, the effectiveness of machine learning algorithms can be compromised. Additionally, farmers must be educated on how to interpret and act on the insights provided by these tools to maximize their utility.
Conclusion
As the agricultural industry continues to evolve, the integration of machine learning for pest and disease detection represents a significant advancement. By leveraging AI-driven tools, farmers can protect their crops in real-time, optimizing resource use and increasing yields. As technology continues to improve, the potential for AI in agriculture will only expand, paving the way for a more sustainable and efficient future in farming.
Keyword: machine learning pest disease detection