Machine Learning for Soil Health Management and Nutrient Optimization
Topic: AI Research Tools
Industry: Agriculture
Discover how machine learning enhances soil health management by optimizing nutrient application for sustainable agriculture and improved crop yields.
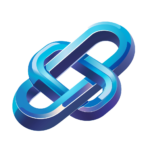
Machine Learning in Soil Health Management: Optimizing Nutrient Application
Introduction to Soil Health Management
Soil health is a critical component of sustainable agriculture, directly influencing crop productivity and environmental quality. Effective soil management practices are essential for optimizing nutrient application, which can enhance yield while minimizing environmental impact. With the advent of artificial intelligence (AI) and machine learning (ML), farmers and agronomists now have powerful tools at their disposal to improve soil health management significantly.
The Role of Machine Learning in Agriculture
Machine learning, a subset of artificial intelligence, involves algorithms that can learn from and make predictions based on data. In the context of agriculture, ML can analyze vast amounts of data related to soil properties, weather patterns, and crop performance. By leveraging these insights, farmers can make informed decisions regarding nutrient application, ultimately leading to healthier soils and more sustainable farming practices.
Implementing AI in Soil Health Management
To effectively integrate AI into soil health management, several key steps can be taken:
- Data Collection: Gathering data from various sources such as soil sensors, satellite imagery, and historical yield data is crucial for training machine learning models.
- Data Analysis: Utilizing ML algorithms to analyze the collected data helps in identifying patterns and correlations that can inform nutrient management strategies.
- Decision Support Systems: Developing AI-driven decision support tools can assist farmers in determining the optimal nutrient application rates based on real-time data and predictive analytics.
Examples of AI-Driven Tools for Nutrient Management
Several innovative tools and products are currently available that harness the power of AI and machine learning for soil health management:
1. SoilOptix
SoilOptix is a precision agriculture tool that utilizes advanced soil mapping technology combined with machine learning. It provides detailed soil nutrient profiles and variability maps, allowing farmers to tailor their nutrient applications based on specific soil conditions. This targeted approach not only enhances crop yield but also reduces excess fertilizer use, promoting environmental sustainability.
2. CropX
CropX is an AI-driven soil sensing platform that integrates soil moisture data and weather forecasts to optimize irrigation and nutrient application. By analyzing soil conditions in real-time, CropX enables farmers to apply the right amount of nutrients at the right time, ensuring that crops receive optimal nourishment while minimizing waste.
3. Agremo
Agremo is an AI-powered agricultural analytics platform that provides insights into crop health and soil conditions. By utilizing aerial imagery and machine learning algorithms, Agremo can assess nutrient deficiencies and recommend corrective actions. This proactive approach helps farmers address soil health issues before they impact crop yields.
Benefits of Machine Learning in Soil Health Management
The integration of machine learning into soil health management offers numerous benefits:
- Enhanced Precision: Machine learning algorithms can analyze complex datasets to provide precise recommendations for nutrient application, reducing the likelihood of over-fertilization.
- Cost Efficiency: By optimizing nutrient use, farmers can lower input costs while maintaining or even increasing crop yields.
- Sustainability: Improved nutrient management contributes to healthier soils and reduces the environmental impact of agriculture, aligning with sustainable farming practices.
Conclusion
As the agricultural sector continues to evolve, the integration of machine learning and AI into soil health management is becoming increasingly vital. By leveraging data-driven insights and innovative tools, farmers can optimize nutrient application, enhance soil health, and contribute to sustainable agricultural practices. The future of farming lies in the intelligent use of technology, and machine learning is at the forefront of this transformation.
Keyword: machine learning soil health management