Machine Learning in Air Quality Monitoring and Pollution Control
Topic: AI Research Tools
Industry: Environmental Sciences
Discover how machine learning enhances air quality monitoring and pollution control through predictive modeling data integration and smart city solutions for a cleaner environment.
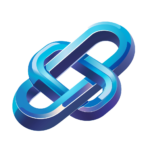
Machine Learning Applications in Air Quality Monitoring and Pollution Control
Introduction to Machine Learning in Environmental Sciences
As the world faces increasing environmental challenges, the integration of machine learning (ML) in air quality monitoring and pollution control has become a pivotal area of research. Machine learning, a subset of artificial intelligence (AI), enables the analysis of vast datasets, allowing for enhanced decision-making and predictive capabilities in environmental sciences.
The Role of AI in Air Quality Monitoring
AI technologies, particularly machine learning algorithms, can process and analyze complex environmental data to identify patterns and trends in air quality. These capabilities are crucial for real-time monitoring and long-term forecasting of air pollution levels.
Predictive Modeling
Machine learning models can be trained on historical air quality data to predict future pollution levels. For instance, algorithms such as regression analysis, decision trees, and neural networks can be employed to forecast air quality based on various factors, including weather conditions, traffic patterns, and industrial emissions.
Data Integration and Analysis
AI tools can integrate data from multiple sources, including satellite imagery, ground-based sensors, and meteorological data. This comprehensive approach allows for a more accurate assessment of air quality. Tools like Google Earth Engine leverage satellite data to monitor air pollution trends over time, providing valuable insights for researchers and policymakers.
Machine Learning Tools for Pollution Control
Several AI-driven products and tools are currently available that facilitate air quality monitoring and pollution control:
1. Air Quality Sensors
Devices such as the Aeroqual Series provide real-time air quality monitoring by utilizing machine learning algorithms to analyze data from various pollutants. These sensors can be deployed in urban areas to track pollution levels and inform local governments for better regulatory decisions.
2. Predictive Analytics Platforms
Platforms like IBM’s Environmental Intelligence Suite employ AI to analyze environmental data and predict pollution events. By using machine learning algorithms, these platforms can help organizations implement proactive measures to mitigate pollution impacts.
3. Smart City Solutions
Smart city initiatives are increasingly incorporating AI for air quality management. For example, the City of Barcelona uses machine learning algorithms to optimize traffic flow, thereby reducing vehicle emissions and improving air quality. These solutions not only enhance urban living conditions but also contribute to sustainability goals.
Challenges and Future Directions
While the potential of machine learning in air quality monitoring and pollution control is significant, several challenges remain. Data quality, privacy concerns, and the need for interdisciplinary collaboration are critical factors that must be addressed to fully harness AI’s capabilities in environmental sciences.
Enhancing Data Quality
Ensuring high-quality data is essential for training effective machine learning models. Collaboration between governmental agencies, research institutions, and private companies can help streamline data collection and sharing processes.
Interdisciplinary Collaboration
The complexity of environmental issues necessitates collaboration among data scientists, environmental scientists, and policymakers. By working together, these stakeholders can develop more robust AI solutions tailored to specific regional challenges.
Conclusion
Machine learning is transforming the landscape of air quality monitoring and pollution control. By leveraging AI-driven tools and technologies, stakeholders can gain valuable insights into pollution patterns and implement effective strategies to combat air quality issues. As research continues to evolve, the potential for machine learning to contribute to a healthier environment is promising.
Keyword: machine learning air quality monitoring