Machine Learning in Energy Trading for Risk Management Efficiency
Topic: AI Productivity Tools
Industry: Energy and Utilities
Discover how machine learning enhances energy trading and risk management by optimizing strategies forecasting prices and improving decision-making for greater profitability.
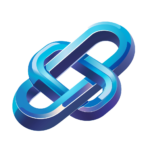
Leveraging Machine Learning for Efficient Energy Trading and Risk Management
Introduction to Machine Learning in Energy Trading
In the rapidly evolving landscape of the energy sector, the integration of artificial intelligence (AI) and machine learning (ML) has become a pivotal strategy for enhancing productivity and operational efficiency. Energy trading and risk management are critical areas where these technologies can significantly impact decision-making processes, optimize trading strategies, and mitigate risks associated with market volatility.Understanding the Role of Machine Learning
Machine learning algorithms analyze vast datasets to identify patterns and trends that human analysts might overlook. In the context of energy trading, ML can be employed to forecast energy prices, assess market conditions, and evaluate the potential risks associated with different trading strategies. By leveraging these insights, companies can make more informed decisions, ultimately leading to improved profitability and reduced risk exposure.Key Applications of Machine Learning in Energy Trading
1. Price Forecasting: Machine learning models can process historical price data, weather patterns, and other relevant variables to predict future energy prices. Tools such as IBM Watson and Google Cloud AI offer robust platforms for developing predictive models that can enhance trading strategies. 2. Risk Assessment: AI-driven risk management solutions, such as those offered by Palantir and RiskMetrics, utilize machine learning algorithms to analyze market data and assess the potential risks associated with various trading scenarios. These tools help traders identify vulnerabilities and make more strategic decisions. 3. Automated Trading Systems: Companies like AutoGrid and GridEdge provide AI-driven platforms that automate trading processes based on predefined algorithms. These systems can execute trades in real-time, optimizing transaction timing and maximizing profit margins.Implementing AI in Energy Trading Strategies
To effectively implement AI and machine learning in energy trading, organizations should consider the following steps: 1. Data Collection and Management: Establish a robust data infrastructure that allows for the collection and storage of relevant datasets. This includes historical trading data, market indicators, and external factors such as weather conditions. 2. Model Development: Utilize AI productivity tools to develop predictive models tailored to specific trading strategies. Platforms like DataRobot and H2O.ai provide user-friendly interfaces for building and deploying machine learning models without extensive coding knowledge. 3. Continuous Monitoring and Optimization: Once models are implemented, continuous monitoring is essential to ensure their accuracy and effectiveness. Tools such as Tableau and Power BI can be used to visualize model performance and make necessary adjustments in real-time.Case Studies of Successful AI Integration
Several organizations have successfully harnessed the power of machine learning in their energy trading operations: – Enel X: This global energy company employs machine learning algorithms to optimize its trading strategies across multiple markets. By analyzing historical data and real-time market conditions, Enel X has improved its forecasting accuracy and reduced trading risks. – BP: BP has integrated AI-driven analytics into its trading operations, allowing for more precise risk assessments and enhanced decision-making capabilities. The company utilizes machine learning to analyze vast amounts of market data, enabling quicker responses to market changes.Challenges and Considerations
While the benefits of leveraging machine learning in energy trading are substantial, organizations must also navigate several challenges: – Data Quality: The effectiveness of machine learning models largely depends on the quality of the input data. Companies must invest in data cleansing and validation processes to ensure accurate outcomes. – Regulatory Compliance: The energy sector is heavily regulated, and organizations need to ensure that their AI implementations comply with relevant laws and regulations. – Skill Gaps: There may be a shortage of skilled professionals who understand both energy trading and machine learning. Companies should consider training existing staff or hiring experts to bridge this gap.Conclusion
The integration of machine learning into energy trading and risk management represents a significant opportunity for organizations in the energy and utilities sector. By leveraging AI-driven tools and platforms, companies can enhance their trading strategies, improve risk assessment capabilities, and ultimately drive greater efficiency and profitability. As the energy landscape continues to evolve, those who embrace these technologies will be well-positioned to thrive in a competitive market.Keyword: machine learning energy trading solutions