Machine Learning in Livestock Management for Better Health
Topic: AI Domain Tools
Industry: Agriculture
Discover how machine learning enhances livestock management by improving animal health and productivity through innovative AI-driven solutions and data insights
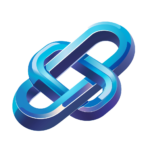
Machine Learning in Livestock Management: Enhancing Animal Health and Productivity
Introduction to Machine Learning in Agriculture
As the agricultural sector faces increasing challenges such as climate change, resource scarcity, and the need for sustainable practices, machine learning (ML) emerges as a transformative technology. In particular, its application in livestock management promises to enhance animal health and productivity through data-driven insights and automation.
The Role of Artificial Intelligence in Livestock Management
Artificial intelligence (AI) can be integrated into various aspects of livestock management, offering solutions that are both innovative and practical. By utilizing AI-driven tools, farmers can monitor animal health, optimize feeding practices, and improve overall farm efficiency. Here are some key areas where machine learning can make a significant impact:
1. Health Monitoring and Disease Prediction
AI technologies can analyze vast amounts of data from livestock, enabling early detection of diseases and health issues. For instance, wearable sensors can track vital signs and behaviors, sending alerts to farmers when anomalies are detected.
Example Tools:
- Moocall: A calving alert system that uses sensors to predict when a cow is about to give birth, reducing the risk of complications.
- Allflex: Offers an animal monitoring system that tracks health metrics and provides real-time data analytics.
2. Feed Optimization
Machine learning algorithms can analyze the nutritional needs of livestock based on various factors such as age, weight, and health status. This data-driven approach allows for the formulation of customized feed plans that enhance growth rates and reduce waste.
Example Tools:
- FeedWatch: A platform that utilizes machine learning to optimize feed rations and improve feed efficiency.
- NutriOpt: An AI-driven tool that provides nutritional recommendations based on real-time data from livestock.
3. Behavioral Analysis
Understanding animal behavior is crucial for effective management. AI can analyze behavioral patterns to identify stressors or changes in activity levels that may indicate health issues or environmental concerns.
Example Tools:
- Smartbow: A system that uses ear tags with sensors to monitor cattle activity and alert farmers to unusual behaviors.
- HerdDogg: This tool uses RFID technology to track livestock movement and behavior, providing insights that can lead to improved herd management.
Implementing AI Solutions in Livestock Management
To effectively implement AI solutions in livestock management, farmers should consider the following steps:
- Data Collection: Invest in technologies that facilitate the collection of relevant data, such as sensors and monitoring devices.
- Integration: Ensure that AI tools can integrate seamlessly with existing farm management systems.
- Training: Provide training for staff to understand and utilize AI tools effectively.
- Continuous Evaluation: Regularly assess the performance of AI tools and make adjustments based on feedback and outcomes.
Conclusion
The integration of machine learning and AI in livestock management is not just a trend; it is a necessity for modern agriculture. By leveraging these technologies, farmers can enhance animal health, improve productivity, and ensure sustainable practices. As the agricultural landscape continues to evolve, embracing AI-driven solutions will be pivotal in meeting the challenges of the future.
Keyword: machine learning livestock management