Machine Learning in Telecom Credit Risk Assessment for 2025
Topic: AI Finance Tools
Industry: Telecommunications
Discover how machine learning transforms credit risk assessment in telecommunications by enhancing accuracy and efficiency for smarter decision-making in 2025.
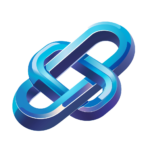
Machine Learning in Telecom Credit Risk Assessment: A 2025 Perspective
Understanding the Need for Credit Risk Assessment in Telecommunications
The telecommunications industry faces unique challenges when it comes to credit risk assessment. As competition intensifies and customer expectations evolve, telecom companies must adopt innovative approaches to evaluate the creditworthiness of their customers effectively. Traditional methods often fall short due to their reliance on historical data and manual processes, which can be both time-consuming and prone to errors. This is where machine learning (ML) comes into play, offering a transformative solution that enhances decision-making processes and improves risk management.The Role of Machine Learning in Credit Risk Assessment
Machine learning algorithms can analyze vast amounts of data at unprecedented speeds, allowing telecom companies to gain insights into customer behavior and credit risk profiles. By leveraging ML, organizations can enhance their predictive capabilities, identify potential risks earlier, and make more informed lending decisions.Key Benefits of Machine Learning in Credit Risk Assessment
- Enhanced Accuracy: Machine learning models can process diverse datasets, including transaction history, customer demographics, and usage patterns, leading to more accurate risk assessments.
- Real-time Analysis: With the ability to analyze data in real-time, telecom companies can quickly respond to changes in customer behavior and market conditions.
- Automated Decision-Making: ML algorithms can automate the credit scoring process, reducing manual intervention and increasing operational efficiency.
- Fraud Detection: Advanced ML techniques can identify anomalies in customer behavior, helping to detect potential fraud before it impacts the business.
AI-Driven Tools and Products for Telecom Credit Risk Assessment
As we look towards 2025, several AI-driven tools and products are poised to revolutionize credit risk assessment in the telecommunications sector. Here are a few notable examples:1. Credit Scoring Models
Telecom companies can employ advanced credit scoring models powered by machine learning. These models utilize a wide range of variables, such as payment history, account usage, and customer interactions, to generate dynamic credit scores. Tools like FICO’s AI-driven credit scoring solutions are already being integrated into telecom operations, providing a more comprehensive view of customer risk.2. Predictive Analytics Platforms
Predictive analytics platforms, such as SAS Viya and IBM Watson Studio, enable telecom companies to build and deploy machine learning models that forecast customer behavior and credit risk. These platforms allow for the integration of various data sources, facilitating a holistic approach to risk assessment.3. Customer Relationship Management (CRM) Systems
Modern CRM systems, like Salesforce Einstein and Microsoft Dynamics 365, incorporate AI capabilities that enhance customer insights. By analyzing customer interactions and transaction data, these systems can help telecom companies identify high-risk customers and tailor their credit offerings accordingly.4. Fraud Detection Solutions
AI-driven fraud detection tools, such as DataRobot and Palantir, leverage machine learning to monitor transactions in real-time, flagging suspicious activities that may indicate credit risk. By employing these solutions, telecom companies can mitigate losses and protect their revenue streams.Implementing Machine Learning in Telecom Credit Risk Assessment
To successfully implement machine learning in credit risk assessment, telecom companies should consider the following steps:1. Data Collection and Integration
Gathering and integrating data from various sources is crucial. This includes customer transaction data, payment histories, and external data such as credit bureau reports. A robust data strategy will lay the foundation for effective machine learning models.2. Model Development and Testing
Developing machine learning models requires collaboration between data scientists and business analysts. It is essential to test these models rigorously to ensure their accuracy and reliability in predicting credit risk.3. Continuous Monitoring and Improvement
Machine learning models are not static; they require continuous monitoring and refinement. Telecom companies should establish feedback loops to assess model performance and make necessary adjustments based on changing market dynamics.Conclusion
As we approach 2025, the integration of machine learning in telecom credit risk assessment will become increasingly vital for companies aiming to stay competitive in a rapidly evolving landscape. By leveraging AI-driven tools and adopting a data-centric approach, telecom organizations can enhance their credit risk assessment processes, reduce losses, and ultimately improve customer satisfaction. The future of telecom finance is undoubtedly intertwined with the advancements in machine learning, paving the way for smarter, more efficient operations.Keyword: machine learning telecom credit risk